Open Access
ARTICLE
EfficientNet-Based Robust Recognition of Peach Plant Diseases in Field Images
1 Department of Computer Science, Islamia College Peshawar, 25120, Pakistan
2 Department of Computer Science, FATA University Kohat, 26100, Pakistan
3 Department of Computer Science, University of Peshawar, 25120, Pakistan
4 Agriculture Research Institute, Mingora, Swat, 19130, Pakistan
* Corresponding Author: Jamil Ahmad. Email:
(This article belongs to the Special Issue: Artificial Intelligence based Smart precision agriculture with analytic pattern in sustainable environments using IoT)
Computers, Materials & Continua 2022, 71(1), 2073-2089. https://doi.org/10.32604/cmc.2022.018961
Received 27 March 2021; Accepted 15 June 2021; Issue published 03 November 2021
Abstract
Plant diseases are a major cause of degraded fruit quality and yield losses. These losses can be significantly reduced with early detection of diseases to ensure their timely treatment, particularly in developing countries. In this regard, an expert system based on deep learning model where the expert knowledge, particularly the one acquired by plant pathologist, is recursively learned by the system and is applied using a smart phone application for use in the target field environment, is being proposed. In this paper, a robust disease detection method is developed based on convolutional neural network (CNN), where its powerful features extraction capabilities are leveraged to detect diseases in images of fruits and leaves. The features extraction pipelines of several state-of-the-art pretrained networks are fine-tuned to achieve optimal detection performance. A novel dataset is collected from peach orchards and extensively augmented using both label-preserving and non-label-preserving transformations. The augmented dataset is used to study the effects of fine-tuning the pretrained networks’ feature extraction pipeline as opposed to keeping the network parameters unchanged. The CNN models, particularly EfficientNet exhibited superior performance on the target dataset once their feature extraction pipelines are fine-tuned. The optimal model is able to achieve 96.6% average accuracy, 90% sensitivity and precision, and 98% specificity on the test set of images.Keywords
Cite This Article
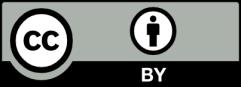