Open Access
ARTICLE
Development of PCCNN-Based Network Intrusion Detection System for EDGE Computing
Department of Computer Science, College of Computer and Information Sciences, Majmaah University, Al-Majmaah 11952, Saudi Arabia
* Corresponding Author: Mohd Abdul Rahim Khan. Email:
(This article belongs to the Special Issue: Green IoT Networks using Machine Learning, Deep Learning Models)
Computers, Materials & Continua 2022, 71(1), 1769-1788. https://doi.org/10.32604/cmc.2022.018708
Received 18 March 2021; Accepted 04 May 2021; Issue published 03 November 2021
Abstract
Intrusion Detection System (IDS) plays a crucial role in detecting and identifying the DoS and DDoS type of attacks on IoT devices. However, anomaly-based techniques do not provide acceptable accuracy for efficacious intrusion detection. Also, we found many difficulty levels when applying IDS to IoT devices for identifying attempted attacks. Given this background, we designed a solution to detect intrusions using the Convolutional Neural Network (CNN) for Enhanced Data rates for GSM Evolution (EDGE) Computing. We created two separate categories to handle the attack and non-attack events in the system. The findings of this study indicate that this approach was significantly effective. We attempted both multiclass and binary classification. In the case of binary, we clustered all malicious traffic data in a single class. Also, we developed 13 layers of Sequential 1-D CNN for IDS detection and assessed them on the public dataset NSL-KDD. Principal Component Analysis (PCA) was implemented to decrease the size of the feature vector based on feature extraction and engineering. The approach proposed in the current investigation obtained accuracies of 99.34% and 99.13% for binary and multiclass classification, respectively, for the NSL-KDD dataset. The experimental outcomes showed that the proposed Principal Component-based Convolution Neural Network (PCCNN) approach achieved greater precision based on deep learning and has potential use in modern intrusion detection for IoT systems.Keywords
Cite This Article
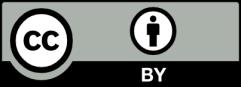
This work is licensed under a Creative Commons Attribution 4.0 International License , which permits unrestricted use, distribution, and reproduction in any medium, provided the original work is properly cited.