Open Access
ARTICLE
Automatic Heart Disease Detection by Classification of Ventricular Arrhythmias on ECG Using Machine Learning
1 Department of Computer Science and Information Technology, University of Sargodha, Sargodha, 40100, Pakistan
2 School of Systems and Technology, University of Management and Technology, Lahore, 54782, Pakistan
3 COMSAT University Islamabad, Wah Campus, Wah Cantt, Pakistan
4 College of Computer Engineering and Sciences, Prince Sattam Bin Abdulaziz University, Al-Khraj, Saudi Arabia
5 Department of ICT Convergence, Soonchunhyang University, Asan, 31538, Korea
6 Department of Computer Science, HITEC University Taxila, Pakistan
* Corresponding Author: Yunyoung Nam. Email:
(This article belongs to the Special Issue: Recent Advances in Deep Learning for Medical Image Analysis)
Computers, Materials & Continua 2022, 71(1), 17-33. https://doi.org/10.32604/cmc.2022.018613
Received 14 March 2021; Accepted 18 April 2021; Issue published 03 November 2021
Abstract
This paper focuses on detecting diseased signals and arrhythmias classification into two classes: ventricular tachycardia and premature ventricular contraction. The sole purpose of the signal detection is used to determine if a signal has been collected from a healthy or sick person. The proposed research approach presents a mathematical model for the signal detector based on calculating the instantaneous frequency (IF). Once a signal taken from a patient is detected, then the classifier takes that signal as input and classifies the target disease by predicting the class label. While applying the classifier, templates are designed separately for ventricular tachycardia and premature ventricular contraction. Similarities of a given signal with both the templates are computed in the spectral domain. The empirical analysis reveals precisions for the detector and the applied classifier are 100% and 77.27%, respectively. Moreover, instantaneous frequency analysis provides a benchmark that IF of a normal signal ranges from 0.8 to 1.1 Hz whereas IF range for ventricular tachycardia and premature ventricular contraction is 0.08–0.6 Hz. This indicates a serious loss of high-frequency contents in the spectrum, implying that the heart’s overall activity is slowed down. This study may help medical practitioners in detecting the heart disease type based on signal analysis.Keywords
Cite This Article
Citations
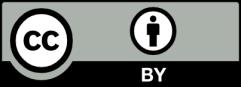