Open Access
ARTICLE
An Integrated Deep Learning Framework for Fruits Diseases Classification
1 Department of Computer Science, HITEC University Taxila, Taxila, Pakistan
2 College of Computer Science and Engineering, University of Ha'il, Ha'il, Saudi Arabia
3 Department of Computer Science, Bahria University, Islamabad
4 College of Computer Engineering and Sciences, Prince Sattam Bin Abdulaziz University, Al-Khraj, Saudi Arabia
5 Department of Computer Science and Engineering, Soonchunhyang University, Asan, Korea
6 Faculty of Applied Computing and Technology, Noroff University College, Kristiansand, Norway
* Corresponding Author: Yunyoung Nam. Email:
(This article belongs to the Special Issue: Recent Advances in Deep Learning and Saliency Methods for Agriculture)
Computers, Materials & Continua 2022, 71(1), 1387-1402. https://doi.org/10.32604/cmc.2022.017701
Received 08 February 2021; Accepted 04 April 2021; Issue published 03 November 2021
Abstract
Agriculture has been an important research area in the field of image processing for the last five years. Diseases affect the quality and quantity of fruits, thereby disrupting the economy of a country. Many computerized techniques have been introduced for detecting and recognizing fruit diseases. However, some issues remain to be addressed, such as irrelevant features and the dimensionality of feature vectors, which increase the computational time of the system. Herein, we propose an integrated deep learning framework for classifying fruit diseases. We consider seven types of fruits, i.e., apple, cherry, blueberry, grapes, peach, citrus, and strawberry. The proposed method comprises several important steps. Initially, data increase is applied, and then two different types of features are extracted. In the first feature type, texture and color features, i.e., classical features, are extracted. In the second type, deep learning characteristics are extracted using a pretrained model. The pretrained model is reused through transfer learning. Subsequently, both types of features are merged using the maximum mean value of the serial approach. Next, the resulting fused vector is optimized using a harmonic threshold-based genetic algorithm. Finally, the selected features are classified using multiple classifiers. An evaluation is performed on the PlantVillage dataset, and an accuracy of 99% is achieved. A comparison with recent techniques indicate the superiority of the proposed method.Keywords
Cite This Article
Citations
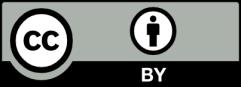