Open Access
ARTICLE
Automatic License Plate Recognition System for Vehicles Using a CNN
1 Department of Computer Science, Chandigarh Group of Colleges, Landran, 140307, India
2 Department of Computer Science, College of Computer Sciences and Information Technology, King Faisal University, Al-Ahsa, Saudi Arabia
3 Department of Research, Innovations, Sponsored Projects & Entrepreneurship, Chandigarh Group of Colleges, Landran, 140307, India
4 Department of Intelligent Mechatronics Engineering, Sejong University, Seoul, 05006, South Korea
* Corresponding Author: Shakeel Ahmed. Email:
Computers, Materials & Continua 2022, 71(1), 35-50. https://doi.org/10.32604/cmc.2022.017681
Received 07 February 2021; Accepted 02 April 2021; Issue published 03 November 2021
Abstract
Automatic License Plate Recognition (ALPR) systems are important in Intelligent Transportation Services (ITS) as they help ensure effective law enforcement and security. These systems play a significant role in border surveillance, ensuring safeguards, and handling vehicle-related crime. The most effective approach for implementing ALPR systems utilizes deep learning via a convolutional neural network (CNN). A CNN works on an input image by assigning significance to various features of the image and differentiating them from each other. CNNs are popular for license plate character recognition. However, little has been reported on the results of these systems with regard to unusual varieties of license plates or their success at night. We present an efficient ALPR system that uses a CNN for character recognition. A combination of pre-processing and morphological operations was applied to enhance input image quality, which aids system efficiency. The system has various features, such as the ability to recognize multi-line, skewed, and multi-font license plates. It also works efficiently in night mode and can be used for different vehicle types. An overall accuracy of 98.13% was achieved using the proposed CNN technique.Keywords
Cite This Article
Citations
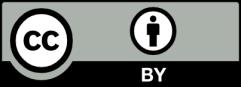
This work is licensed under a Creative Commons Attribution 4.0 International License , which permits unrestricted use, distribution, and reproduction in any medium, provided the original work is properly cited.