Open Access
ARTICLE
Comparative Study of Transfer Learning Models for Retinal Disease Diagnosis from Fundus Images
1 Department of ICT Convergence, Soonchunhyang University, Asan, 31538, Korea
2 Department of Ophthalmology, Soonchunhyang University Bucheon Hospital, Soonchunhyang University College of Medicine, Bucheon, 420-767, Korea
3 Department of Computer Science and Engineering, Soonchunhyang University, Asan, 31538, Korea
* Corresponding Author: Yunyoung Nam. Email:
(This article belongs to the Special Issue: Future Generation of Artificial Intelligence and Intelligent Internet of Things)
Computers, Materials & Continua 2022, 70(3), 5821-5834. https://doi.org/10.32604/cmc.2022.021943
Received 21 July 2021; Accepted 25 August 2021; Issue published 11 October 2021
Abstract
While the usage of digital ocular fundus image has been widespread in ophthalmology practice, the interpretation of the image has been still on the hands of the ophthalmologists which are quite costly. We explored a robust deep learning system that detects three major ocular diseases: diabetic retinopathy (DR), glaucoma (GLC), and age-related macular degeneration (AMD). The proposed method is composed of two steps. First, an initial quality evaluation in the classification system is proposed to filter out poor-quality images to enhance its performance, a technique that has not been explored previously. Second, the transfer learning technique is used with various convolutional neural networks (CNN) models that automatically learn a thousand features in the digital retinal image, and are based on those features for diagnosing eye diseases. Comparison performance of many models is conducted to find the optimal model which fits with fundus classification. Among the different CNN models, DenseNet-201 outperforms others with an area under the receiver operating characteristic curve of 0.99. Furthermore, the corresponding specificities for healthy, DR, GLC, and AMD patients are found to be 89.52%, 96.69%, 89.58%, and 100%, respectively. These results demonstrate that the proposed method can reduce the time-consumption by automatically diagnosing multiple eye diseases using computer-aided assistance tools.Keywords
Cite This Article
K. Pin, J. Ho Chang and Y. Nam, "Comparative study of transfer learning models for retinal disease diagnosis from fundus images," Computers, Materials & Continua, vol. 70, no.3, pp. 5821–5834, 2022. https://doi.org/10.32604/cmc.2022.021943Citations
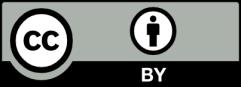