Open Access
ARTICLE
Deep Q-Learning Based Optimal Query Routing Approach for Unstructured P2P Network
1 Department of Computer Science, Faculty of Science at Al Dawadmi, Shaqra University, Shaqra, Saudi Arabia
* Corresponding Author: Abdullah Shawan Alotaibi. Email:
(This article belongs to the Special Issue: Emerging Trends in Software-Defined Networking for Industry 4.0)
Computers, Materials & Continua 2022, 70(3), 5765-5781. https://doi.org/10.32604/cmc.2022.021941
Received 21 July 2021; Accepted 22 August 2021; Issue published 11 October 2021
Abstract
Deep Reinforcement Learning (DRL) is a class of Machine Learning (ML) that combines Deep Learning with Reinforcement Learning and provides a framework by which a system can learn from its previous actions in an environment to select its efforts in the future efficiently. DRL has been used in many application fields, including games, robots, networks, etc. for creating autonomous systems that improve themselves with experience. It is well acknowledged that DRL is well suited to solve optimization problems in distributed systems in general and network routing especially. Therefore, a novel query routing approach called Deep Reinforcement Learning based Route Selection (DRLRS) is proposed for unstructured P2P networks based on a Deep Q-Learning algorithm. The main objective of this approach is to achieve better retrieval effectiveness with reduced searching cost by less number of connected peers, exchanged messages, and reduced time. The simulation results shows a significantly improve searching a resource with compression to k-Random Walker and Directed BFS. Here, retrieval effectiveness, search cost in terms of connected peers, and average overhead are 1.28, 106, 149, respectively.Keywords
Cite This Article
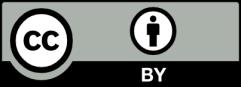
This work is licensed under a Creative Commons Attribution 4.0 International License , which permits unrestricted use, distribution, and reproduction in any medium, provided the original work is properly cited.