Open Access
ARTICLE
An Intelligent Forecasting Model for Disease Prediction Using Stack Ensembling Approach
1 Computer Science & Engineering Department, Dr. B.R. Ambedkar National Institute of Technology, Jalandhar, India
2 Computer Science & Engineering Department, Lovely Professional University, Jalandhar, India
3 Department of Information Technology, College of Computers and Information Technology, Taif University, P. O. Box 11099, Taif 21944, Saudi Arabia
4 Department of Computer Science, College of Computers and Information Technology, Taif University, P. O. Box 11099, Taif 21944, Saudi Arabia
5 Chitkara University Institute of Engineering and Technology, Chitkara University, Punjab, India
* Corresponding Author: Nitin Goyal. Email:
Computers, Materials & Continua 2022, 70(3), 6041-6055. https://doi.org/10.32604/cmc.2022.021747
Received 12 July 2021; Accepted 13 August 2021; Issue published 11 October 2021
Abstract
This research work proposes a new stack-based generalization ensemble model to forecast the number of incidences of conjunctivitis disease. In addition to forecasting the occurrences of conjunctivitis incidences, the proposed model also improves performance by using the ensemble model. Weekly rate of acute Conjunctivitis per 1000 for Hong Kong is collected for the duration of the first week of January 2010 to the last week of December 2019. Pre-processing techniques such as imputation of missing values and logarithmic transformation are applied to pre-process the data sets. A stacked generalization ensemble model based on Auto-ARIMA (Autoregressive Integrated Moving Average), NNAR (Neural Network Autoregression), ETS (Exponential Smoothing), HW (Holt Winter) is proposed and applied on the dataset. Predictive analysis is conducted on the collected dataset of conjunctivitis disease, and further compared for different performance measures. The result shows that the RMSE (Root Mean Square Error), MAE (Mean Absolute Error), MAPE (Mean Absolute Percentage Error), ACF1 (Auto Correlation Function) of the proposed ensemble is decreased significantly. Considering the RMSE, for instance, error values are reduced by 39.23%, 9.13%, 20.42%, and 17.13% in comparison to Auto-ARIMA, NAR, ETS, and HW model respectively. This research concludes that the accuracy of the forecasting of diseases can be significantly increased by applying the proposed stack generalization ensemble model as it minimizes the prediction error and hence provides better prediction trends as compared to Auto-ARIMA, NAR, ETS, and HW model applied discretelyKeywords
Cite This Article
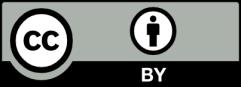
This work is licensed under a Creative Commons Attribution 4.0 International License , which permits unrestricted use, distribution, and reproduction in any medium, provided the original work is properly cited.