Open Access
ARTICLE
Deep Learning Based Intelligent Industrial Fault Diagnosis Model
1 Center for Artificial Intelligence & Research (CAIR), Chennai Institute of Technology, Chennai, 600069, India
2 Al-Nahrain Nanorenewable Energy Research Center, Al-Nahrain University, Baghdad, 64074, Iraq
3 COMBA I+D Research Group of Universidad Santiago de Cali, Santiago de Cali, Colombia
* Corresponding Author: R. Surendran. Email:
Computers, Materials & Continua 2022, 70(3), 6323-6338. https://doi.org/10.32604/cmc.2022.021716
Received 12 July 2021; Accepted 18 August 2021; Issue published 11 October 2021
Abstract
In the present industrial revolution era, the industrial mechanical system becomes incessantly highly intelligent and composite. So, it is necessary to develop data-driven and monitoring approaches for achieving quick, trustable, and high-quality analysis in an automated way. Fault diagnosis is an essential process to verify the safety and reliability operations of rotating machinery. The advent of deep learning (DL) methods employed to diagnose faults in rotating machinery by extracting a set of feature vectors from the vibration signals. This paper presents an Intelligent Industrial Fault Diagnosis using Sailfish Optimized Inception with Residual Network (IIFD-SOIR) Model. The proposed model operates on three major processes namely signal representation, feature extraction, and classification. The proposed model uses a Continuous Wavelet Transform (CWT) is for preprocessed representation of the original vibration signal. In addition, Inception with ResNet v2 based feature extraction model is applied to generate high-level features. Besides, the parameter tuning of Inception with the ResNet v2 model is carried out using a sailfish optimizer. Finally, a multilayer perceptron (MLP) is applied as a classification technique to diagnose the faults proficiently. Extensive experimentation takes place to ensure the outcome of the presented model on the gearbox dataset and a motor bearing dataset. The experimental outcome indicated that the IIFD-SOIR model has reached a higher average accuracy of 99.6% and 99.64% on the applied gearbox dataset and bearing dataset. The simulation outcome ensured that the proposed model has attained maximum performance over the compared methods.Keywords
Cite This Article
Citations
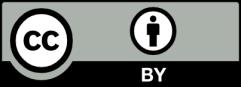
This work is licensed under a Creative Commons Attribution 4.0 International License , which permits unrestricted use, distribution, and reproduction in any medium, provided the original work is properly cited.