Open Access
ARTICLE
Robust Length of Stay Prediction Model for Indoor Patients
1 Department of Mechatronics and Control Engineering, University of Engineering and Technology, Lahore, 54000, Pakistan
2 Department of Information Sciences, Division of Science and Technology, University of Education, Lahore, 54000, Pakistan
3 Department of Computer Science and Information, College of Science in Zulfi, Majmaah University, Al-Majmaah, 11952, Saudi Arabia
4 Riphah School of Computing & Innovation, Faculty of Computing, Riphah International University, Lahore Campus, Lahore, 54000, Pakistan
5 Pattern Recognition and Machine Learning Lab, Department of Software, Gachon University, Seongnam, 13557, Korea
* Corresponding Author: Muhammad Adnan Khan. Email:
Computers, Materials & Continua 2022, 70(3), 5519-5536. https://doi.org/10.32604/cmc.2022.021666
Received 08 July 2021; Accepted 09 August 2021; Issue published 11 October 2021
Abstract
Due to unforeseen climate change, complicated chronic diseases, and mutation of viruses’ hospital administration’s top challenge is to know about the Length of stay (LOS) of different diseased patients in the hospitals. Hospital management does not exactly know when the existing patient leaves the hospital; this information could be crucial for hospital management. It could allow them to take more patients for admission. As a result, hospitals face many problems managing available resources and new patients in getting entries for their prompt treatment. Therefore, a robust model needs to be designed to help hospital administration predict patients’ LOS to resolve these issues. For this purpose, a very large-sized data (more than 2.3 million patients’ data) related to New-York Hospitals patients and containing information about a wide range of diseases including Bone-Marrow, Tuberculosis, Intestinal Transplant, Mental illness, Leukaemia, Spinal cord injury, Trauma, Rehabilitation, Kidney and Alcoholic Patients, HIV Patients, Malignant Breast disorder, Asthma, Respiratory distress syndrome, etc. have been analyzed to predict the LOS. We selected six Machine learning (ML) models named: Multiple linear regression (MLR), Lasso regression (LR), Ridge regression (RR), Decision tree regression (DTR), Extreme gradient boosting regression (XGBR), and Random Forest regression (RFR). The selected models’ predictive performance was checked using R square and Mean square error (MSE) as the performance evaluation criteria. Our results revealed the superior predictive performance of the RFR model, both in terms of RS score (92%) and MSE score (5), among all selected models. By Exploratory data analysis (EDA), we conclude that maximum stay was between 0 to 5 days with the meantime of each patient 5.3 days and more than 50 years old patients spent more days in the hospital. Based on the average LOS, results revealed that the patients with diagnoses related to birth complications spent more days in the hospital than other diseases. This finding could help predict the future length of hospital stay of new patients, which will help the hospital administration estimate and manage their resources efficiently.Keywords
Cite This Article
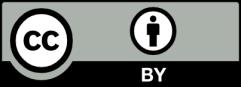
This work is licensed under a Creative Commons Attribution 4.0 International License , which permits unrestricted use, distribution, and reproduction in any medium, provided the original work is properly cited.