Open Access
ARTICLE
Estimating Fuel-Efficient Air Plane Trajectories Using Machine Learning
1 Chitkara University Institute of Engineering & Technology, Chitkara University, Punjab, 140401, India
2 Department of Software, Sejong University, Seoul, 05006, Korea
3 College of Technological Innovation, Zayed University, UAE
4 School of Electrical Engineering, Korea University, Seoul, Korea
* Corresponding Author: Sangheon Pack. Email:
(This article belongs to the Special Issue: Machine Learning Empowered Secure Computing for Intelligent Systems)
Computers, Materials & Continua 2022, 70(3), 6189-6204. https://doi.org/10.32604/cmc.2022.021657
Received 09 July 2021; Accepted 23 August 2021; Issue published 11 October 2021
Abstract
Airline industry has witnessed a tremendous growth in the recent past. Percentage of people choosing air travel as first choice to commute is continuously increasing. Highly demanding and congested air routes are resulting in inadvertent delays, additional fuel consumption and high emission of greenhouse gases. Trajectory planning involves creation identification of cost-effective flight plans for optimal utilization of fuel and time. This situation warrants the need of an intelligent system for dynamic planning of optimized flight trajectories with least human intervention required. In this paper, an algorithm for dynamic planning of optimized flight trajectories has been proposed. The proposed algorithm divides the airspace into four dimensional cubes and calculate a dynamic score for each cube to cumulatively represent estimated weather, aerodynamic drag and air traffic within that virtual cube. There are several constraints like simultaneous flight separation rules, weather conditions like air temperature, pressure, humidity, wind speed and direction that pose a real challenge for calculating optimal flight trajectories. To validate the proposed methodology, a case analysis was undertaken within Indian airspace. The flight routes were simulated for four different air routes within Indian airspace. The experiment results observed a seven percent reduction in drag values on the predicted path, hence indicates reduction in carbon footprint and better fuel economy.Keywords
Cite This Article
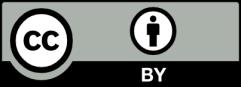