Open Access
ARTICLE
IoT-Cloud Empowered Aerial Scene Classification for Unmanned Aerial Vehicles
1 Department of Information Technology, Sri Sivasubramaniya Nadar College of Engineering, Chennai, 603110, India
2 Department of Information Technology, QIS College of Engineering & Technology, Ongole, 523001, India
3 Department of Electronics and Communications Engineering, Saranathan College of Engineering, Trichy, 620012, India
4 Department of Electrical and Electronics Engineering, SRM Institute of Science and Technology, Chennai, 603203, India
5 Department of Entrepreneurship and Logistics, Plekhanov Russian University of Economics, Moscow, 117997, Russia
6 Department of Logistics, State University of Management, Moscow, 109542, Russia
7 Department of Computer Science, University of Oviedo, Oviedo, 33003, Spain
* Corresponding Author: K. R. Uthayan. Email:
Computers, Materials & Continua 2022, 70(3), 5161-5177. https://doi.org/10.32604/cmc.2022.021300
Received 28 June 2021; Accepted 29 July 2021; Issue published 11 October 2021
Abstract
Recent trends in communication technologies and unmanned aerial vehicles (UAVs) find its application in several areas such as healthcare, surveillance, transportation, etc. Besides, the integration of Internet of things (IoT) with cloud computing environment offers several benefits for the UAV communication. At the same time, aerial scene classification is one of the major research areas in UAV-enabled MEC systems. In UAV aerial imagery, efficient image representation is crucial for the purpose of scene classification. The existing scene classification techniques generate mid-level image features with limited representation capabilities that often end up in producing average results. Therefore, the current research work introduces a new DL-enabled aerial scene classification model for UAV-enabled MEC systems. The presented model enables the UAVs to capture aerial images which are then transmitted to MEC for further processing. Next, Capsule Network (CapsNet)-based feature extraction technique is applied to derive a set of useful feature vectors from the aerial image. It is important to have an appropriate hyperparameter tuning strategy, since manual parameter tuning of DL model tend to produce several configuration errors. In order to achieve this and to determine the hyperparameters of CapsNet model, Shuffled Shepherd Optimization (SSO) algorithm is implemented. Finally, Backpropagation Neural Network (BPNN) classification model is applied to determine the appropriate class labels of aerial images. The performance of SSO-CapsNet model was validated against two openly-accessible datasets namely, UC Merced (UCM) Land Use dataset and WHU-RS dataset. The proposed SSO-CapsNet model outperformed the existing state-of-the-art methods and achieved maximum accuracy of 0.983, precision of 0.985, recall of 0.982, and F-score of 0.983.Keywords
Cite This Article
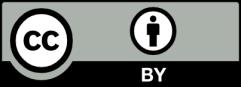
This work is licensed under a Creative Commons Attribution 4.0 International License , which permits unrestricted use, distribution, and reproduction in any medium, provided the original work is properly cited.