Open Access
ARTICLE
Using Capsule Networks for Android Malware Detection Through Orientation-Based Features
1 Deanship of Preparatory Year and Supporting Studies, Computer Science Department, Imam Abdulrahman BinFaisal University, Dammam, Saudi Arabia
2 National University of Computer and Emerging Sciences, Pakistan
3 Department of Computer Science, Islamic University of Medina, Medina, Saudi Arabia
* Corresponding Author: Sohail Khan. Email:
Computers, Materials & Continua 2022, 70(3), 5345-5362. https://doi.org/10.32604/cmc.2022.021271
Received 28 June 2021; Accepted 30 July 2021; Issue published 11 October 2021
Abstract
Mobile phones are an essential part of modern life. The two popular mobile phone platforms, Android and iPhone Operating System (iOS), have an immense impact on the lives of millions of people. Among these two, Android currently boasts more than 84% market share. Thus, any personal data put on it are at great risk if not properly protected. On the other hand, more than a million pieces of malware have been reported on Android in just 2021 till date. Detecting and mitigating all this malware is extremely difficult for any set of human experts. Due to this reason, machine learning–and specifically deep learning–has been utilized in the recent past to resolve this issue. However, deep learning models have primarily been designed for image analysis. While this line of research has shown promising results, it has been difficult to really understand what the features extracted by deep learning models are in the domain of malware. Moreover, due to the translation invariance property of popular models based on Convolutional Neural Network (CNN), the true potential of deep learning for malware analysis is yet to be realized. To resolve this issue, we envision the use of Capsule Networks (CapsNets), a state-of-the-art model in deep learning. We argue that since CapsNets are orientation-based in terms of images, they can potentially be used to capture spatial relationships between different features at different locations within a sequence of opcodes. We design a deep learning-based architecture that efficiently and effectively handles very large scale malware datasets to detect Android malware without resorting to very deep networks. This leads to much faster detection as well as increased accuracy. We achieve state-of-the-art F1 score of 0.987 with an FPR of just 0.002 for three very large, real-world malware datasets. Our code is made available as open source and can be used to further enhance our work with minimal effort.Keywords
Cite This Article
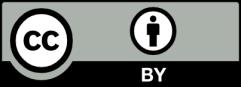
This work is licensed under a Creative Commons Attribution 4.0 International License , which permits unrestricted use, distribution, and reproduction in any medium, provided the original work is properly cited.