Open Access
ARTICLE
Fusion of Infrared and Visible Images Using Fuzzy Based Siamese Convolutional Network
1 Department of Electrical Engineering, National Taipei University of Technology, Taipei, 10608, Taiwan
2 Department Virtualization, School of Computer Science, University of Petroleum & Energy Studies, Dehradun, India
3 College of Computer Science and Information technology, King Faisal University, 36362, Saudi Arabia
4 College of Computing and Informatics, Saudi Electronic University, Riyadh, 11673, Saudi Arabia
* Corresponding Author: Deepika Koundal. Email:
(This article belongs to the Special Issue: Innovations in Artificial Intelligence using Data Mining and Big Data)
Computers, Materials & Continua 2022, 70(3), 5503-5518. https://doi.org/10.32604/cmc.2022.021125
Received 24 June 2021; Accepted 03 August 2021; Issue published 11 October 2021
Abstract
Traditional techniques based on image fusion are arduous in integrating complementary or heterogeneous infrared (IR)/visible (VS) images. Dissimilarities in various kind of features in these images are vital to preserve in the single fused image. Hence, simultaneous preservation of both the aspects at the same time is a challenging task. However, most of the existing methods utilize the manual extraction of features; and manual complicated designing of fusion rules resulted in a blurry artifact in the fused image. Therefore, this study has proposed a hybrid algorithm for the integration of multi-features among two heterogeneous images. Firstly, fuzzification of two IR/VS images has been done by feeding it to the fuzzy sets to remove the uncertainty present in the background and object of interest of the image. Secondly, images have been learned by two parallel branches of the siamese convolutional neural network (CNN) to extract prominent features from the images as well as high-frequency information to produce focus maps containing source image information. Finally, the obtained focused maps which contained the detailed integrated information are directly mapped with the source image via pixel-wise strategy to result in fused image. Different parameters have been used to evaluate the performance of the proposed image fusion by achieving 1.008 for mutual information (MI), 0.841 for entropy , 0.655 for edge information (EI), 0.652 for human perception (HP), and 0.980 for image structural similarity (ISS). Experimental results have shown that the proposed technique has attained the best qualitative and quantitative results using 78 publically available images in comparison to the existing discrete cosine transform (DCT), anisotropic diffusion & karhunen-loeve (ADKL), guided filter (GF), random walk (RW), principal component analysis (PCA), and convolutional neural network (CNN) methods.Keywords
Cite This Article
Citations
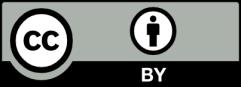
This work is licensed under a Creative Commons Attribution 4.0 International License , which permits unrestricted use, distribution, and reproduction in any medium, provided the original work is properly cited.