Open Access
ARTICLE
Intelligent Deep Learning Based Automated Fish Detection Model for UWSN
1 Department of Natural and Applied Sciences, College of Community - Aflaj, Prince Sattam bin Abdulaziz University, Saudi Arabia
2 Department of Information Systems, College of Computer and Information Sciences, Princess Nourah bint Abdulrahman University, Saudi Arabia
3 Department of Computer Science, King Khalid University, Muhayel Aseer, Saudi Arabia & Faculty of Computer and IT, Sana’a University, Sana’a, Yemen
4 Department of Information Systems, King Khalid University, Muhayel Aseer, Saudi Arabia
5 Department of Computer and Self Development, Preparatory Year Deanship, Prince Sattam bin Abdulaziz University, AlKharj, Saudi Arabia
* Corresponding Author: Anwer Mustafa Hilal. Email:
Computers, Materials & Continua 2022, 70(3), 5871-5887. https://doi.org/10.32604/cmc.2022.021093
Received 22 June 2021; Accepted 30 July 2021; Issue published 11 October 2021
Abstract
An exponential growth in advanced technologies has resulted in the exploration of Ocean spaces. It has paved the way for new opportunities that can address questions relevant to diversity, uniqueness, and difficulty of marine life. Underwater Wireless Sensor Networks (UWSNs) are widely used to leverage such opportunities while these networks include a set of vehicles and sensors to monitor the environmental conditions. In this scenario, it is fascinating to design an automated fish detection technique with the help of underwater videos and computer vision techniques so as to estimate and monitor fish biomass in water bodies. Several models have been developed earlier for fish detection. However, they lack robustness to accommodate considerable differences in scenes owing to poor luminosity, fish orientation, structure of seabed, aquatic plant movement in the background and distinctive shapes and texture of fishes from different genus. With this motivation, the current research article introduces an Intelligent Deep Learning based Automated Fish Detection model for UWSN, named IDLAFD-UWSN model. The presented IDLAFD-UWSN model aims at automatic detection of fishes from underwater videos, particularly in blurred and crowded environments. IDLAFD-UWSN model makes use of Mask Region Convolutional Neural Network (Mask RCNN) with Capsule Network as a baseline model for fish detection. Besides, in order to train Mask RCNN, background subtraction process using Gaussian Mixture Model (GMM) model is applied. This model makes use of motion details of fishes in video which consequently integrates the outcome with actual image for the generation of fish-dependent candidate regions. Finally, Wavelet Kernel Extreme Learning Machine (WKELM) model is utilized as a classifier model. The performance of the proposed IDLAFD-UWSN model was tested against benchmark underwater video dataset and the experimental results achieved by IDLAFD-UWSN model were promising in comparison with other state-of-the-art methods under different aspects with the maximum accuracy of 98% and 97% on the applied blurred and crowded datasets respectively.Keywords
Cite This Article
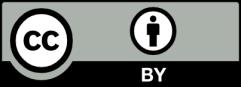
This work is licensed under a Creative Commons Attribution 4.0 International License , which permits unrestricted use, distribution, and reproduction in any medium, provided the original work is properly cited.