Open Access
ARTICLE
A Novel Auto-Annotation Technique for Aspect Level Sentiment Analysis
1 Department of Computer Sciences, Bahria University, Lahore Campus, 54000, Pakistan
2 Computer and Information Science Department, University Teknologi, Petronas, 32610, Malaysia
* Corresponding Author: Muhammad Aasim Qureshi. Email:
(This article belongs to the Special Issue: Machine Learning Empowered Secure Computing for Intelligent Systems)
Computers, Materials & Continua 2022, 70(3), 4987-5004. https://doi.org/10.32604/cmc.2022.020544
Received 27 May 2021; Accepted 28 June 2021; Issue published 11 October 2021
Abstract
In machine learning, sentiment analysis is a technique to find and analyze the sentiments hidden in the text. For sentiment analysis, annotated data is a basic requirement. Generally, this data is manually annotated. Manual annotation is time consuming, costly and laborious process. To overcome these resource constraints this research has proposed a fully automated annotation technique for aspect level sentiment analysis. Dataset is created from the reviews of ten most popular songs on YouTube. Reviews of five aspects—voice, video, music, lyrics and song, are extracted. An N-Gram based technique is proposed. Complete dataset consists of 369436 reviews that took 173.53 s to annotate using the proposed technique while this dataset might have taken approximately 2.07 million seconds (575 h) if it was annotated manually. For the validation of the proposed technique, a sub-dataset—Voice, is annotated manually as well as with the proposed technique. Cohen's Kappa statistics is used to evaluate the degree of agreement between the two annotations. The high Kappa value (i.e., 0.9571%) shows the high level of agreement between the two. This validates that the quality of annotation of the proposed technique is as good as manual annotation even with far less computational cost. This research also contributes in consolidating the guidelines for the manual annotation process.Keywords
Cite This Article
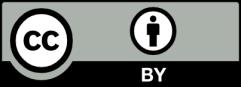
This work is licensed under a Creative Commons Attribution 4.0 International License , which permits unrestricted use, distribution, and reproduction in any medium, provided the original work is properly cited.