Open Access
ARTICLE
EEG-Based Neonatal Sleep Stage Classification Using Ensemble Learning
1 School of Computing, Edinburgh Napier University, Edinburgh EH10 5DT, UK
2 Center for Intelligent Medical Electronics, Department of Electronic Engineering, Fudan University, 200433, China
3 Department of Computer Engineering, Jeju National University, Jejusi, Jeju Special Self-Governing Province, 63243, Korea
* Corresponding Author: Wei Chen. Email:
(This article belongs to the Special Issue: Advancements in Lightweight AI for Constrained Internet of Things Devices for Smart Cities)
Computers, Materials & Continua 2022, 70(3), 4619-4633. https://doi.org/10.32604/cmc.2022.020318
Received 19 May 2021; Accepted 15 July 2021; Issue published 11 October 2021
Abstract
Sleep stage classification can provide important information regarding neonatal brain development and maturation. Visual annotation, using polysomnography (PSG), is considered as a gold standard for neonatal sleep stage classification. However, visual annotation is time consuming and needs professional neurologists. For this reason, an internet of things and ensemble-based automatic sleep stage classification has been proposed in this study. 12 EEG features, from 9 bipolar channels, were used to train and test the base classifiers including convolutional neural network, support vector machine, and multilayer perceptron. Bagging and stacking ensembles are then used to combine the outputs for final classification. The proposed algorithm can reach a mean kappa of 0.73 and 0.66 for 2-stage and 3-stage (wake, active sleep, and quiet sleep) classification, respectively. The proposed network works as a semi-real time application because a smoothing filter is used to hold the sleep stage for 3 min. The high-performance parameters and its ability to work in semi real-time makes it a promising candidate for use in hospitalized newborn infants.Keywords
Cite This Article
Citations
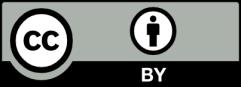
This work is licensed under a Creative Commons Attribution 4.0 International License , which permits unrestricted use, distribution, and reproduction in any medium, provided the original work is properly cited.