Open Access
ARTICLE
An Efficient CNN-Based Hybrid Classification and Segmentation Approach for COVID-19 Detection
1 Department of Information Technology, College of Computer and Information Sciences, Princess Nourah Bint Abdulrahman University, Riyadh 84428, Saudi Arabia
2 Department of Electronics and Electrical Communications, Faculty of Electronic Engineering, Menoufia University, Menouf 32952, Egypt
3 Department of Industrial Electronics and Control Engineering, Faculty of Electronic Engineering, Menoufia University, Menouf 32952, Egypt
4 Department of Electronics and Communications Engineering, Faculty of Engineering, Zagazig University, Zagazig, Sharqia 44519, Egypt
* Corresponding Author: Abeer D. Algarni. Email:
Computers, Materials & Continua 2022, 70(3), 4393-4410. https://doi.org/10.32604/cmc.2022.020265
Received 18 May 2021; Accepted 29 June 2021; Issue published 11 October 2021
Abstract
COVID-19 remains to proliferate precipitously in the world. It has significantly influenced public health, the world economy, and the persons’ lives. Hence, there is a need to speed up the diagnosis and precautions to deal with COVID-19 patients. With this explosion of this pandemic, there is a need for automated diagnosis tools to help specialists based on medical images. This paper presents a hybrid Convolutional Neural Network (CNN)-based classification and segmentation approach for COVID-19 detection from Computed Tomography (CT) images. The proposed approach is employed to classify and segment the COVID-19, pneumonia, and normal CT images. The classification stage is firstly applied to detect and classify the input medical CT images. Then, the segmentation stage is performed to distinguish between pneumonia and COVID-19 CT images. The classification stage is implemented based on a simple and efficient CNN deep learning model. This model comprises four Rectified Linear Units (ReLUs), four batch normalization layers, and four convolutional (Conv) layers. The Conv layer depends on filters with sizes of 64, 32, 16, and 8. A 2 × 2 window and a stride of 2 are employed in the utilized four max-pooling layers. A soft-max activation function and a Fully-Connected (FC) layer are utilized in the classification stage to perform the detection process. For the segmentation process, the Simplified Pulse Coupled Neural Network (SPCNN) is utilized in the proposed hybrid approach. The proposed segmentation approach is based on salient object detection to localize the COVID-19 or pneumonia region, accurately. To summarize the contributions of the paper, we can say that the classification process with a CNN model can be the first stage a highly-effective automated diagnosis system. Once the images are accepted by the system, it is possible to perform further processing through a segmentation process to isolate the regions of interest in the images. The region of interest can be assesses both automatically and through experts. This strategy helps so much in saving the time and efforts of specialists with the explosion of COVID-19 pandemic in the world. The proposed classification approach is applied for different scenarios of 80%, 70%, or 60% of the data for training and 20%, 30, or 40% of the data for testing, respectively. In these scenarios, the proposed approach achieves classification accuracies of 100%, 99.45%, and 98.55%, respectively. Thus, the obtained results demonstrate and prove the efficacy of the proposed approach for assisting the specialists in automated medical diagnosis services.Keywords
Cite This Article
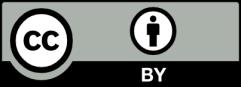
This work is licensed under a Creative Commons Attribution 4.0 International License , which permits unrestricted use, distribution, and reproduction in any medium, provided the original work is properly cited.