Open Access
ARTICLE
Efficient Morphological Segmentation of Brain Hemorrhage Stroke Lesion Through MultiResUNet
1 Department of Biomedical Instrumentation Engineering, Avinashilingam Institute for Home Science and Higher Education for Women, Coimbatore, India
2 Department of ECE, Karpagam College of Engineering, Coimbatore, India
* Corresponding Author: R. Shijitha. Email:
Computers, Materials & Continua 2022, 70(3), 5233-5249. https://doi.org/10.32604/cmc.2022.020227
Received 15 May 2021; Accepted 02 July 2021; Issue published 11 October 2021
Abstract
Brain Hemorrhagic stroke is a serious malady that is caused by the drop in blood flow through the brain and causes the brain to malfunction. Precise segmentation of brain hemorrhage is crucial, so an enhanced segmentation is carried out in this research work. The brain image of various patients has taken using an MRI scanner by the utilization of T1, T2, and FLAIR sequence. This work aims to segment the Brain Hemorrhagic stroke using deep learning-based Multi-resolution UNet (multires UNet) through morphological operations. It is hard to precisely segment the brain lesions to extract the existing region of stroke. This crucial step is accomplished by this proposed MMU-Net methodology by precise segmentation of stroke lesions. The proposed method efficiently determines the hemorrhagic stroke with improved accuracy of 95% compared with the existing segmentation techniques such as U-net++, ResNet, Multires UNET and 3D-ResU-Net and also provides improved performance of 2D and 3D U-Net with an enhanced outcome. The performance measure of the proposed methodology acquires an improved accuracy, precision ratio, sensitivity, and specificity rate of 0.07%, 0.04%, 0.04%, and 0.05% in comparison to U-net, ResNet, Multires UNET and 3D-ResU-Net techniques respectively.Keywords
Cite This Article
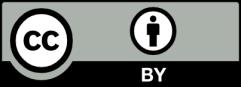