Open Access
ARTICLE
Machine Learning Approaches to Detect DoS and Their Effect on WSNs Lifetime
1 College of Computing and Informatics, Saudi Electronic University, Riyadh, 11673, Saudi Arabia
2 The School of Information Technology, Sebha University, Sebha, 71, Libya
* Corresponding Author: Rami Ahmad. Email:
(This article belongs to the Special Issue: Advanced IoT Industrial Solutions and Cyber Security Threats in Communication Networks)
Computers, Materials & Continua 2022, 70(3), 4922-4946. https://doi.org/10.32604/cmc.2022.020044
Received 07 May 2021; Accepted 08 July 2021; Issue published 11 October 2021
Abstract
Energy and security remain the main two challenges in Wireless Sensor Networks (WSNs). Therefore, protecting these WSN networks from Denial of Service (DoS) and Distributed DoS (DDoS) is one of the WSN networks security tasks. Traditional packet deep scan systems that rely on open field inspection in transport layer security packets and the open field encryption trend are making machine learning-based systems the only viable choice for these types of attacks. This paper contributes to the evaluation of the use machine learning algorithms in WSN nodes traffic and their effect on WSN network life time. We examined the performance metrics of different machine learning classification categories such as K-Nearest Neighbour (KNN), Logistic Regression (LR), Support Vector Machine (SVM), Gboost, Decision Tree (DT), Naïve Bayes, Long Short Term Memory (LSTM), and Multi-Layer Perceptron (MLP) on a WSN-dataset in different sizes. The test results proved that the statistical and logical classification categories performed the best on numeric statistical datasets, and the Gboost algorithm showed the best performance compared to different algorithms on average of all performance metrics. The performance metrics used in these validations were accuracy, F1-score, False Positive Ratio (FPR), False Negative Ratio (FNR), and the training execution time. Moreover, the test results showed the Gboost algorithm got 99.6%, 98.8%, 0.4% 0.13% in accuracy, F1-score, FPR, and FNR, respectively. At training execution time, it obtained 1.41 s for the average of all training time execution datasets. In addition, this paper demonstrated that for the numeric statistical data type, the best results are in the size of the dataset ranging from 3000 to 6000 records and the percentage between categories is not less than 50% for each category with the other categories. Furthermore, this paper investigated the effect of Gboost on the WSN lifetime, which resulted in a 32% reduction compared to other Gboost-free scenarios.Keywords
Cite This Article
Citations
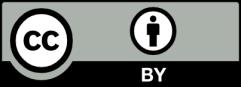
This work is licensed under a Creative Commons Attribution 4.0 International License , which permits unrestricted use, distribution, and reproduction in any medium, provided the original work is properly cited.