Open Access
ARTICLE
Towards Securing Machine Learning Models Against Membership Inference Attacks
1 Departement of STIC, Higher Institute of Technological Studies of Gabes, General Directorate of Technological Studies, Rades, 2098, Tunisia
2 Research Team on Intelligent Machines, National Engineering School of Gabes, Gabes University, Gabes, 6072, Tunisia
3 SERCOM-Lab., Tunisia Polytechnic School, Carthage University, Tunis, 1054, Tunisia
4 Department of IT, College of Computing and Informatics, Saudi Electronic University, Medina, 42376, Saudi Arabia
5 School of Science, Engineering and Environment, University of Salford, Manchester, M5 4WT, UK
6 Department of Science, College of Science and Theoretical Studies, Saudi Electronic University, Riyadh, 11673, Saudi Arabia
7INSAT, SERCOM-Lab., Tunisia Polytechnic School, Carthage University, Tunis, 1080, Tunisia
* Corresponding Author: Sana Belguith. Email:
(This article belongs to the Special Issue: AI for Wearable Sensing--Smartphone / Smartwatch User Identification / Authentication)
Computers, Materials & Continua 2022, 70(3), 4897-4919. https://doi.org/10.32604/cmc.2022.019709
Received 23 April 2021; Accepted 20 June 2021; Issue published 11 October 2021
Abstract
From fraud detection to speech recognition, including price prediction, Machine Learning (ML) applications are manifold and can significantly improve different areas. Nevertheless, machine learning models are vulnerable and are exposed to different security and privacy attacks. Hence, these issues should be addressed while using ML models to preserve the security and privacy of the data used. There is a need to secure ML models, especially in the training phase to preserve the privacy of the training datasets and to minimise the information leakage. In this paper, we present an overview of ML threats and vulnerabilities, and we highlight current progress in the research works proposing defence techniques against ML security and privacy attacks. The relevant background for the different attacks occurring in both the training and testing/inferring phases is introduced before presenting a detailed overview of Membership Inference Attacks (MIA) and the related countermeasures. In this paper, we introduce a countermeasure against membership inference attacks (MIA) on Conventional Neural Networks (CNN) based on dropout and L2 regularization. Through experimental analysis, we demonstrate that this defence technique can mitigate the risks of MIA attacks while ensuring an acceptable accuracy of the model. Indeed, using CNN model training on two datasets CIFAR-10 and CIFAR-100, we empirically verify the ability of our defence strategy to decrease the impact of MIA on our model and we compare results of five different classifiers. Moreover, we present a solution to achieve a trade-off between the performance of the model and the mitigation of MIA attack.Keywords
Cite This Article
Citations
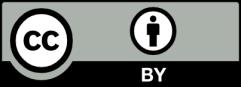
This work is licensed under a Creative Commons Attribution 4.0 International License , which permits unrestricted use, distribution, and reproduction in any medium, provided the original work is properly cited.