Open Access
ARTICLE
A Non-Destructive Time Series Model for the Estimation of Cherry Coffee Production
1 Telematics Engineering Group, University of Cauca, Popayán, 190002, Colombia
2 INRAE, University of Toulouse, UMR 1248 AGIR, F-31326, Castanet Tolosan, France
3 Department of Software Engineering, University of Granada, Granada, 18071, Spain
* Corresponding Author: Jhonn Pablo Rodríguez. Email:
(This article belongs to the Special Issue: Artificial Intelligence based Smart precision agriculture with analytic pattern in sustainable environments using IoT)
Computers, Materials & Continua 2022, 70(3), 4725-4743. https://doi.org/10.32604/cmc.2022.019135
Received 03 April 2021; Accepted 07 May 2021; Issue published 11 October 2021
Abstract
Coffee plays a key role in the generation of rural employment in Colombia. More than 785,000 workers are directly employed in this activity, which represents the 26% of all jobs in the agricultural sector. Colombian coffee growers estimate the production of cherry coffee with the main aim of planning the required activities, and resources (number of workers, required infrastructures), anticipating negotiations, estimating, price, and foreseeing losses of coffee production in a specific territory. These important processes can be affected by several factors that are not easy to predict (e.g., weather variability, diseases, or plagues.). In this paper, we propose a non-destructive time series model, based on weather and crop management information, that estimate coffee production allowing coffee growers to improve their management of agricultural activities such as flowering calendars, harvesting seasons, definition of irrigation methods, nutrition calendars, and programming the times of concentration of production to define the amount of personnel needed for harvesting. The combination of time series and machine learning algorithms based on regression trees (XGBOOST, TR and RF) provides very positive results for the test dataset collected in real conditions for more than a year. The best results were obtained by the XGBOOST model (MAE = 0.03; RMSE = 0.01), and a difference of approximately 0.57% absolute to the main harvest of 2018.Keywords
Cite This Article
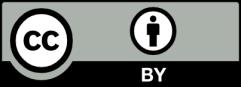