Open Access
ARTICLE
Ensembles of Deep Learning Framework for Stomach Abnormalities Classification
Department of Artificial Intelligence, Faculty of Computer Science and Information Technology, Universiti Malaya, 50603 Kuala Lumpur, Malaysia
* Corresponding Author: Chu Kiong Loo. Email:
(This article belongs to the Special Issue: Recent Advances in Deep Learning for Medical Image Analysis)
Computers, Materials & Continua 2022, 70(3), 4357-4372. https://doi.org/10.32604/cmc.2022.019076
Received 01 April 2021; Accepted 18 June 2021; Issue published 11 October 2021
Abstract
Abnormalities of the gastrointestinal tract are widespread worldwide today. Generally, an effective way to diagnose these life-threatening diseases is based on endoscopy, which comprises a vast number of images. However, the main challenge in this area is that the process is time-consuming and fatiguing for a gastroenterologist to examine every image in the set. Thus, this led to the rise of studies on designing AI-based systems to assist physicians in the diagnosis. In several medical imaging tasks, deep learning methods, especially convolutional neural networks (CNNs), have contributed to the state-of-the-art outcomes, where the complicated nonlinear relation between target classes and data can be learned and not limit to hand-crafted features. On the other hand, hyperparameters are commonly set manually, which may take a long time and leave the risk of non-optimal hyperparameters for classification. An effective tool for tuning optimal hyperparameters of deep CNN is Bayesian optimization. However, due to the complexity of the CNN, the network can be regarded as a black-box model where the information stored within it is hard to interpret. Hence, Explainable Artificial Intelligence (XAI) techniques are applied to overcome this issue by interpreting the decisions of the CNNs in such wise the physicians can trust. To play an essential role in real-time medical diagnosis, CNN-based models need to be accurate and interpretable, while the uncertainty must be handled. Therefore, a novel method comprising of three phases is proposed to classify these life-threatening diseases. At first, hyperparameter tuning is performed using Bayesian optimization for two state-of-the-art deep CNNs, and then Darknet53 and InceptionV3 features are extracted from these fine-tunned models. Secondly, XAI techniques are used to interpret which part of the images CNN takes for feature extraction. At last, the features are fused, and uncertainties are handled by selecting entropy-based features. The experimental results show that the proposed method outperforms existing methods by achieving an accuracy of 97% based on a Bayesian optimized Support Vector Machine classifier.
Keywords
Cite This Article
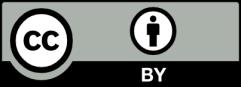
This work is licensed under a Creative Commons Attribution 4.0 International License , which permits unrestricted use, distribution, and reproduction in any medium, provided the original work is properly cited.