Open Access
ARTICLE
An Improved Convolutional Neural Network Model for DNA Classification
1 Department of Information Technology, College of Computer and Information Sciences, Princess Nourah Bint Abdulrahman University, Riyadh, Saudi Arabia
2 Department of Electronics and Electrical Communications Engineering, Faculty of Electronic Engineering, Menoufia University, Menoufia, 32952, Egypt
3 Department of Computer Science and Engineering, Faculty of Electronic Engineering, Menoufia University, Menoufia, 32952, Egypt
* Corresponding Author:Naglaa. F. Soliman. Email:
Computers, Materials & Continua 2022, 70(3), 5907-5927. https://doi.org/10.32604/cmc.2022.018860
Received 24 March 2021; Accepted 11 June 2021; Issue published 11 October 2021
Abstract
Recently, deep learning (DL) became one of the essential tools in bioinformatics. A modified convolutional neural network (CNN) is employed in this paper for building an integrated model for deoxyribonucleic acid (DNA) classification. In any CNN model, convolutional layers are used to extract features followed by max-pooling layers to reduce the dimensionality of features. A novel method based on downsampling and CNNs is introduced for feature reduction. The downsampling is an improved form of the existing pooling layer to obtain better classification accuracy. The two-dimensional discrete transform (2D DT) and two-dimensional random projection (2D RP) methods are applied for downsampling. They convert the high-dimensional data to low-dimensional data and transform the data to the most significant feature vectors. However, there are parameters which directly affect how a CNN model is trained. In this paper, some issues concerned with the training of CNNs have been handled. The CNNs are examined by changing some hyperparameters such as the learning rate, size of minibatch, and the number of epochs. Training and assessment of the performance of CNNs are carried out on 16S rRNA bacterial sequences. Simulation results indicate that the utilization of a CNN based on wavelet subsampling yields the best trade-off between processing time and accuracy with a learning rate equal to 0.0001, a size of minibatch equal to 64, and a number of epochs equal to 20.
Keywords
Cite This Article
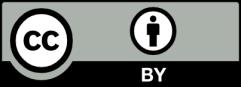
This work is licensed under a Creative Commons Attribution 4.0 International License , which permits unrestricted use, distribution, and reproduction in any medium, provided the original work is properly cited.