Open Access
A Hybrid Neural Network and Box-Jenkins Models for Time Series Forecasting
1 Department of Information Technology, College of Computer, Qassim University, Buraydah, Saudi Arabia
2 Department of Computer Science, College of Applied Sciences, Taiz University, Taiz, Yemen
3 Intelligent Analytics Group (IAG), College of Computer, Qassim University, Buraydah, Saudi Arabia
4 Faculty of Computer Sciences and Information Systems, Thamar University, Thamar, Yemen
5 Faculty of Administration Science, Ibb University, Ibb, Yemen
6 Department of Computer Science, College of Computer, Qassim University, Buraydah, Saudi Arabia
* Corresponding Author:Mohammed Hadwan. Email:
Computers, Materials & Continua 2022, 70(3), 4829-4845. https://doi.org/10.32604/cmc.2022.017824
Received 13 February 2021; Accepted 28 July 2021; Issue published 11 October 2021
Abstract
Time series forecasting plays a significant role in numerous applications, including but not limited to, industrial planning, water consumption, medical domains, exchange rates and consumer price index. The main problem is insufficient forecasting accuracy. The present study proposes a hybrid forecasting methods to address this need. The proposed method includes three models. The first model is based on the autoregressive integrated moving average (ARIMA) statistical model; the second model is a back propagation neural network (BPNN) with adaptive slope and momentum parameters; and the third model is a hybridization between ARIMA and BPNN (ARIMA/BPNN) and artificial neural networks and ARIMA (ARIMA/ANN) to gain the benefits of linear and nonlinear modeling. The forecasting models proposed in this study are used to predict the indices of the consumer price index (CPI), and predict the expected number of cancer patients in the Ibb Province in Yemen. Statistical standard measures used to evaluate the proposed method include (i) mean square error, (ii) mean absolute error, (iii) root mean square error, and (iv) mean absolute percentage error. Based on the computational results, the improvement rate of forecasting the CPI dataset was 5%, 71%, and 4% for ARIMA/BPNN model, ARIMA/ANN model, and BPNN model respectively; while the result for cancer patients’ dataset was 7%, 200%, and 19% for ARIMA/BPNN model, ARIMA/ANN model, and BPNN model respectively. Therefore, it is obvious that the proposed method reduced the randomness degree, and the alterations affected the time series with data non-linearity. The ARIMA/ANN model outperformed each of its components when it was applied separately in terms of increasing the accuracy of forecasting and decreasing the overall errors of forecasting.
Keywords
Cite This Article
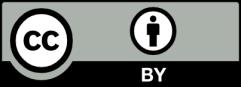