Open Access
ARTICLE
Convolutional Neural Network-Based Regression for Predicting the Chloride Ion Diffusion Coefficient of Concrete
1 Architectural Engineering, Hanyang University ERICA, Ansan, 15588, Korea
2 Graduate School of Information, Yonsei University, Seoul, 03722, Korea
3 Division of Smart Convergence Engineering, Hanyang University ERICA, Ansan, 15588, Korea
* Corresponding Author: Sang Hyo Lee. Email:
Computers, Materials & Continua 2022, 70(3), 5059-5071. https://doi.org/10.32604/cmc.2022.017262
Received 25 January 2021; Accepted 30 July 2021; Issue published 11 October 2021
Abstract
The durability performance of reinforced concrete (RC) building structures is significantly affected by the corrosion of the steel reinforcement due to chloride penetration, thus, the chloride ion diffusion coefficient should be investigated through experiments or theoretical equations to assess the durability of an RC structure. This study aims to predict the chloride ion diffusion coefficient of concrete, a heterogeneous material. A convolutional neural network (CNN)-based regression model that learns the condition of the concrete surface through deep learning, is developed to efficiently obtain the chloride ion diffusion coefficient. For the model implementation to determine the chloride ion diffusion coefficient, concrete mixes with w/c ratios of 0.33, 0.40, 0.46, 0.50, 0.62, and 0.68, are cured for 28 days; subsequently, the surface image data of the specimens are collected. Finally, the proposed model predicts the chloride ion diffusion coefficient using the concrete surface image data and exhibits an error of approximately 1.5E−12 /s. The results suggest the applicability of proposed model to the field of facility maintenance for estimating the chloride ion diffusion coefficient of concrete using images.Keywords
Cite This Article
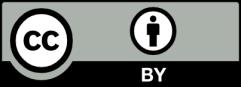
This work is licensed under a Creative Commons Attribution 4.0 International License , which permits unrestricted use, distribution, and reproduction in any medium, provided the original work is properly cited.