Open Access
A Global Training Model for Beat Classification Using Basic Electrocardiogram Morphological Features
1 School of Information Technology, Deakin University, Burwood, VIC, 3128, Australia
2 Industrial Engineering Department, College of Engineering, King Saud University, P.O. Box 800, Riyadh, 11421, Saudi Arabia
* Corresponding Author: Shafiq Ahmad. Email:
(This article belongs to the Special Issue: Deep Learning and Parallel Computing for Intelligent and Efficient IoT)
Computers, Materials & Continua 2022, 70(3), 4503-4521. https://doi.org/10.32604/cmc.2022.015474
Received 23 November 2020; Accepted 17 July 2021; Issue published 11 October 2021
Abstract
Clinical Study and automatic diagnosis of electrocardiogram (ECG) data always remain a challenge in diagnosing cardiovascular activities. The analysis of ECG data relies on various factors like morphological features, classification techniques, methods or models used to diagnose and its performance improvement. Another crucial factor in the methodology is how to train the model for each patient. Existing approaches use standard training model which faces challenges when training data has variation due to individual patient characteristics resulting in a lower detection accuracy. This paper proposes an adaptive approach to identify performance improvement in building a training model that analyze global training methodology against an individual training methodology and identifying a gap between them. We provide our investigation and comparative study on these methods and model with standard classification techniques with basic morphological features and Heart Rate Variability (HRV) that may aid real time application. This approach helps in analyzing and evaluating the performance of different techniques and can suggests adoption of a best model identification with efficient technique and efficient attribute set for real-time systems.
Keywords
Cite This Article
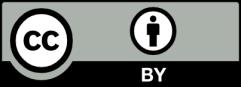
This work is licensed under a Creative Commons Attribution 4.0 International License , which permits unrestricted use, distribution, and reproduction in any medium, provided the original work is properly cited.