Open Access
ARTICLE
Prediction of Extremist Behaviour and Suicide Bombing from Terrorism Contents Using Supervised Learning
Department of Computer Science, University of Engineering and Technology, 54890, Lahore
* Corresponding Author: Nasir Mahmood. Email:
(This article belongs to the Special Issue: Machine Learning-based Intelligent Systems: Theories, Algorithms, and Applications)
Computers, Materials & Continua 2022, 70(3), 4411-4428. https://doi.org/10.32604/cmc.2022.013956
Received 30 August 2020; Accepted 16 March 2021; Issue published 11 October 2021
Abstract
This study proposes an architecture for the prediction of extremist human behaviour from projected suicide bombings. By linking ‘dots’ of police data comprising scattered information of people, groups, logistics, locations, communication, and spatiotemporal characters on different social media groups, the proposed architecture will spawn beneficial information. This useful information will, in turn, help the police both in predicting potential terrorist events and in investigating previous events. Furthermore, this architecture will aid in the identification of criminals and their associates and handlers. Terrorism is psychological warfare, which, in the broadest sense, can be defined as the utilisation of deliberate violence for economic, political or religious purposes. In this study, a supervised learning-based approach was adopted to develop the proposed architecture. The dataset was prepared from the suicide bomb blast data of Pakistan obtained from the South Asia Terrorism Portal (SATP). As the proposed architecture was simulated, the supervised learning-based classifiers naïve Bayes and Hoeffding Tree reached 72.17% accuracy. One of the additional benefits this study offers is the ability to predict the target audience of potential suicide bomb blasts, which may be used to eliminate future threats or, at least, minimise the number of casualties and other property losses.Keywords
Cite This Article
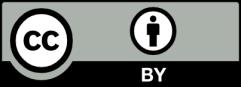
This work is licensed under a Creative Commons Attribution 4.0 International License , which permits unrestricted use, distribution, and reproduction in any medium, provided the original work is properly cited.