Open Access
ARTICLE
Price Prediction of Seasonal Items Using Machine Learning and Statistical Methods
Faculty of Computers and Informatics, Zagazig University, Zagazig, Egypt
* Corresponding Author: Ahmad Salah. Email:
Computers, Materials & Continua 2022, 70(2), 3473-3489. https://doi.org/10.32604/cmc.2022.020782
Received 07 June 2021; Accepted 08 July 2021; Issue published 27 September 2021
Abstract
Price prediction of goods is a vital point of research due to how common e-commerce platforms are. There are several efforts conducted to forecast the price of items using classic machine learning algorithms and statistical models. These models can predict prices of various financial instruments, e.g., gold, oil, cryptocurrencies, stocks, and second-hand items. Despite these efforts, the literature has no model for predicting the prices of seasonal goods (e.g., Christmas gifts). In this context, we framed the task of seasonal goods price prediction as a regression problem. First, we utilized a real online trailer dataset of Christmas gifts and then we proposed several machine learning-based models and one statistical-based model to predict the prices of these seasonal products. Second, we utilized a real-life dataset of Christmas gifts for the prediction task. Then, we proposed support vector regressor (SVR), linear regression, random forest, and ridge models as machine learning models for price prediction. Next, we proposed an autoregressive-integrated-moving-average (ARIMA) model for the same purpose as a statistical-based model. Finally, we evaluated the performance of the proposed models; the comparison shows that the best performing model was the random forest model, followed by the ARIMA model.Keywords
Cite This Article
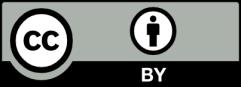
This work is licensed under a Creative Commons Attribution 4.0 International License , which permits unrestricted use, distribution, and reproduction in any medium, provided the original work is properly cited.