Open Access
ARTICLE
Optimal Deep Convolution Neural Network for Cervical Cancer Diagnosis Model
1 Department of Medical Equipment Technology, College of Applied Medical Sciences, Majmaah University, Al Majmaah, 11952, Saudi Arabia
2 Faculty of Applied Computing and Technology, Noroff University College, Kristiansand, 4608, Norway
3 Research Group of Embedded Systems and Mobile Application in Health Science, College of Arts, Media and Technology, Chiang Mai University, Chiang Mai, 50200, Thailand
* Corresponding Author: Orawit Thinnukool. Email:
Computers, Materials & Continua 2022, 70(2), 3295-3309. https://doi.org/10.32604/cmc.2022.020713
Received 03 June 2021; Accepted 04 July 2021; Issue published 27 September 2021
Abstract
Biomedical imaging is an effective way of examining the internal organ of the human body and its diseases. An important kind of biomedical image is Pap smear image that is widely employed for cervical cancer diagnosis. Cervical cancer is a vital reason for increased women’s mortality rate. Proper screening of pap smear images is essential to assist the earlier identification and diagnostic process of cervical cancer. Computer-aided systems for cancerous cell detection need to be developed using deep learning (DL) approaches. This study introduces an intelligent deep convolutional neural network for cervical cancer detection and classification (IDCNN-CDC) model using biomedical pap smear images. The proposed IDCNN-CDC model involves four major processes such as preprocessing, segmentation, feature extraction, and classification. Initially, the Gaussian filter (GF) technique is applied to enhance data through noise removal process in the Pap smear image. The Tsallis entropy technique with the dragonfly optimization (TE-DFO) algorithm determines the segmentation of an image to identify the diseased portions properly. The cell images are fed into the DL based SqueezeNet model to extract deep-learned features. Finally, the extracted features from SqueezeNet are applied to the weighted extreme learning machine (ELM) classification model to detect and classify the cervix cells. For experimental validation, the Herlev database is employed. The database was developed at Herlev University Hospital (Denmark). The experimental outcomes make sure that higher performance of the proposed technique interms of sensitivity, specificity, accuracy, and F-Score.Keywords
Cite This Article
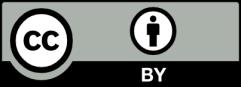
This work is licensed under a Creative Commons Attribution 4.0 International License , which permits unrestricted use, distribution, and reproduction in any medium, provided the original work is properly cited.