Open Access
ARTICLE
HARTIV: Human Activity Recognition Using Temporal Information in Videos
1 CSE Department, G. H. Raisoni Institute of Engineering and Technology, SPPU University, Pune, India
2 CSE Department, Bennett University, Greater Noida, India
3 TML Business Services Limited, Pune, India
4 CSE Department, Anand International College of Engineering, Jaipur, Rajasthan, India
5 College of Industrial Engineering, King Khalid University, Abha, Saudi Arabia
6 Faculty of Computers and Information, South Valley University, Qena, 83523, Egypt
* Corresponding Author: Hammam Alshazly. Email:
(This article belongs to the Special Issue: Recent Advances in Metaheuristic Techniques and Their Real-World Applications)
Computers, Materials & Continua 2022, 70(2), 3919-3938. https://doi.org/10.32604/cmc.2022.020655
Received 02 June 2021; Accepted 12 July 2021; Issue published 27 September 2021
Abstract
Nowadays, the most challenging and important problem of computer vision is to detect human activities and recognize the same with temporal information from video data. The video datasets are generated using cameras available in various devices that can be in a static or dynamic position and are referred to as untrimmed videos. Smarter monitoring is a historical necessity in which commonly occurring, regular, and out-of-the-ordinary activities can be automatically identified using intelligence systems and computer vision technology. In a long video, human activity may be present anywhere in the video. There can be a single or multiple human activities present in such videos. This paper presents a deep learning-based methodology to identify the locally present human activities in the video sequences captured by a single wide-view camera in a sports environment. The recognition process is split into four parts: firstly, the video is divided into different set of frames, then the human body part in a sequence of frames is identified, next process is to identify the human activity using a convolutional neural network and finally the time information of the observed postures for each activity is determined with the help of a deep learning algorithm. The proposed approach has been tested on two different sports datasets including ActivityNet and THUMOS. Three sports activities like swimming, cricket bowling and high jump have been considered in this paper and classified with the temporal information i.e., the start and end time for every activity present in the video. The convolutional neural network and long short-term memory are used for feature extraction of temporal action recognition from video data of sports activity. The outcomes show that the proposed method for activity recognition in the sports domain outperforms the existing methods.Keywords
Cite This Article
Citations
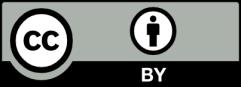