Open Access
ARTICLE
Artifacts Reduction Using Multi-Scale Feature Attention Network in Compressed Medical Images
Department of Convergence IT Engineering, Kyungnam University, Changwon, 51767, Korea
* Corresponding Author: Dongsan Jun. Email:
(This article belongs to the Special Issue: Integrity and Multimedia Data Management in Healthcare Applications using IoT)
Computers, Materials & Continua 2022, 70(2), 3267-3279. https://doi.org/10.32604/cmc.2022.020651
Received 01 June 2021; Accepted 03 July 2021; Issue published 27 September 2021
Abstract
Medical image compression is one of the essential technologies to facilitate real-time medical data transmission in remote healthcare applications. In general, image compression can introduce undesired coding artifacts, such as blocking artifacts and ringing effects. In this paper, we proposed a Multi-Scale Feature Attention Network (MSFAN) with two essential parts, which are multi-scale feature extraction layers and feature attention layers to efficiently remove coding artifacts of compressed medical images. Multi-scale feature extraction layers have four Feature Extraction (FE) blocks. Each FE block consists of five convolution layers and one CA block for weighted skip connection. In order to optimize the proposed network architectures, a variety of verification tests were conducted using validation dataset. We used Computer Vision Center-Clinic Database (CVC-ClinicDB) consisting of 612 colonoscopy medical images to evaluate the enhancement of image restoration. The proposed MSFAN can achieve improved PSNR gains as high as 0.25 and 0.24 dB on average compared to DnCNN and DCSC, respectively.Keywords
Cite This Article
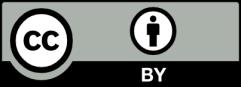
This work is licensed under a Creative Commons Attribution 4.0 International License , which permits unrestricted use, distribution, and reproduction in any medium, provided the original work is properly cited.