Open Access
ARTICLE
Optimization of Cognitive Radio System Using Self-Learning Salp Swarm Algorithm
1 Department of Electronics & Communication Engineering, Chandigarh University, Mohali, 140413, India
2 School of VLSI Design and Embedded Systems, National Institute of Technology, Kurukshetra, 136119, India
3 School of Electronics & Communication Engineering, Shri Mata Vaishno Devi University, Katra, 182320, India
4 Department of Computer Science, College of Computers and Information Technology, Taif University, Taif, 21944, Saudi Arabia
5 Department of Computer Engineering, College of Computer and Information Technology, Taif University, Taif, 21994, Saudi Arabia
6 Department of Mathematics, Faculty of Science, Mansoura University, Mansoura, 35516, Egypt
7 Department of Computational Mathematics, Science, and Engineering (CMSE), Michigan State University, East Lansing, MI, 48824, USA
* Corresponding Author: Mohamed Abouhawwash. Email:
Computers, Materials & Continua 2022, 70(2), 3821-3835. https://doi.org/10.32604/cmc.2022.020592
Received 30 May 2021; Accepted 08 July 2021; Issue published 27 September 2021
Abstract
Cognitive Radio (CR) has been developed as an enabling technology that allows the unused or underused spectrum to be used dynamically to increase spectral efficiency. To improve the overall performance of the CR system it is extremely important to adapt or reconfigure the system parameters. The Decision Engine is a major module in the CR-based system that not only includes radio monitoring and cognition functions but also responsible for parameter adaptation. As meta-heuristic algorithms offer numerous advantages compared to traditional mathematical approaches, the performance of these algorithms is investigated in order to design an efficient CR system that is able to adapt the transmitting parameters to effectively reduce power consumption, bit error rate and adjacent interference of the channel, while maximized secondary user throughput. Self-Learning Salp Swarm Algorithm (SLSSA) is a recent meta-heuristic algorithm that is the enhanced version of SSA inspired by the swarming behavior of salps. In this work, the parametric adaption of CR system is performed by SLSSA and the simulation results show that SLSSA has high accuracy, stability and outperforms other competitive algorithms for maximizing the throughput of secondary users. The results obtained with SLSSA are also shown to be extremely satisfactory and need fewer iterations to converge compared to the competitive methods.Keywords
Cite This Article
Citations
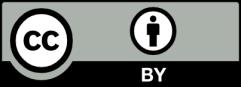