Open Access
ARTICLE
AI Based Traffic Flow Prediction Model for Connected and Autonomous Electric Vehicles
1 Department of Electrical and Electronics Engineering, University College of Engineering, Panruti, 607106, India
2 Department of Electronics and Communication Engineering, Koneru Lakshmaiah Education Foundation, Vijayawada, 522502, India
3 Centre for Nanoelectronics and VLSI Design, SENSE, VIT University, Chennai Campus, Chennai, 600127, India
4 Department of Electronics and Communication Engineering, K. Ramakrishnan College of Engineering, Tiruchirappalli, 621112, India
5 Department of Electronics and Communication Engineering, Kalasalingam Academy of Research and Education, Krishnankoil, 626126, India
6 Department of Entrepreneurship and Logistics, Plekhanov Russian University of Economics, Moscow, 117997, Russia
7 Department of Logistics, State University of Management, Moscow, 109542, Russia
* Corresponding Author: P. Thamizhazhagan. Email:
Computers, Materials & Continua 2022, 70(2), 3333-3347. https://doi.org/10.32604/cmc.2022.020197
Received 14 May 2021; Accepted 15 June 2021; Issue published 27 September 2021
Abstract
There is a paradigm shift happening in automotive industry towards electric vehicles as environment and sustainability issues gained momentum in the recent years among potential users. Connected and Autonomous Electric Vehicle (CAEV) technologies are fascinating the automakers and inducing them to manufacture connected autonomous vehicles with self-driving features such as autopilot and self-parking. Therefore, Traffic Flow Prediction (TFP) is identified as a major issue in CAEV technologies which needs to be addressed with the help of Deep Learning (DL) techniques. In this view, the current research paper presents an artificial intelligence-based parallel autoencoder for TFP, abbreviated as AIPAE-TFP model in CAEV. The presented model involves two major processes namely, feature engineering and TFP. In feature engineering process, there are multiple stages involved such as feature construction, feature selection, and feature extraction. In addition to the above, a Support Vector Data Description (SVDD) model is also used in the filtration of anomaly points and smoothen the raw data. Finally, AIPAE model is applied to determine the predictive values of traffic flow. In order to illustrate the proficiency of the model’s predictive outcomes, a set of simulations was performed and the results were investigated under distinct aspects. The experimentation outcomes verified the effectual performance of the proposed AIPAE-TFP model over other methods.Keywords
Cite This Article
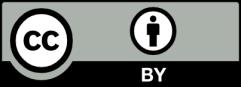
This work is licensed under a Creative Commons Attribution 4.0 International License , which permits unrestricted use, distribution, and reproduction in any medium, provided the original work is properly cited.