Open Access
ARTICLE
Data Fusion-Based Machine Learning Architecture for Intrusion Detection
1 Pattern Recognition and Machine Learning Lab, Department of Software, Gachon University, Seongnam, 13557, Korea
2 Center for Cyber Security, Faculty of Information Science and Technology, Universiti Kebansaan Malaysia (UKM), 43600, Bangi, Selangor, Malaysia
3 School of Information Technology, Skyline University College, University City Sharjah, 1797, Sharjah, UAE
4 School of Computer Science, National College of Business Administration & Economics, Lahore, 54000, Pakistan
* Corresponding Author: Sang-Woong Lee. Email:
Computers, Materials & Continua 2022, 70(2), 3399-3413. https://doi.org/10.32604/cmc.2022.020173
Received 12 May 2021; Accepted 29 June 2021; Issue published 27 September 2021
Abstract
In recent years, the infrastructure of Wireless Internet of Sensor Networks (WIoSNs) has been more complicated owing to developments in the internet and devices’ connectivity. To effectively prepare, control, hold and optimize wireless sensor networks, a better assessment needs to be conducted. The field of artificial intelligence has made a great deal of progress with deep learning systems and these techniques have been used for data analysis. This study investigates the methodology of Real Time Sequential Deep Extreme Learning Machine (RTS-DELM) implemented to wireless Internet of Things (IoT) enabled sensor networks for the detection of any intrusion activity. Data fusion is a well-known methodology that can be beneficial for the improvement of data accuracy, as well as for the maximizing of wireless sensor networks lifespan. We also suggested an approach that not only makes the casting of parallel data fusion network but also render their computations more effective. By using the Real Time Sequential Deep Extreme Learning Machine (RTS-DELM) methodology, an excessive degree of reliability with a minimal error rate of any intrusion activity in wireless sensor networks is accomplished. Simulation results show that wireless sensor networks are optimized effectively to monitor and detect any malicious or intrusion activity through this proposed approach. Eventually, threats and a more general outlook are explored.Keywords
Cite This Article
Citations
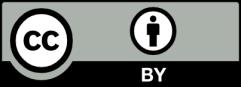
This work is licensed under a Creative Commons Attribution 4.0 International License , which permits unrestricted use, distribution, and reproduction in any medium, provided the original work is properly cited.