Open Access
ARTICLE
Deep Rank-Based Average Pooling Network for Covid-19 Recognition
1 School of Mathematics and Actuarial Science, University of Leicester, LE1 7RH, UK
2 Department of Computer Science, HITEC University Taxila, Taxila, Pakistan
3 Department of Biomedical Engineering, Kalasalingam Academy of Research and Education, 626 126, Tamil Nadu, India
4 Department of Computer Science, Design & Journalism, Creighton University, Omaha, Nebraska, USA
5 Science in Civil Engineering, University of Florida, Gainesville, Florida, FL 32608, USA
6 School of Informatics, University of Leicester, UK
* Corresponding Author: Yu-Dong Zhang. Email:
(This article belongs to the Special Issue: Recent Advances in Deep Learning and Saliency Methods for Agriculture)
Computers, Materials & Continua 2022, 70(2), 2797-2813. https://doi.org/10.32604/cmc.2022.020140
Received 11 May 2021; Accepted 18 June 2021; Issue published 27 September 2021
Abstract
(Aim) To make a more accurate and precise COVID-19 diagnosis system, this study proposed a novel deep rank-based average pooling network (DRAPNet) model, i.e., deep rank-based average pooling network, for COVID-19 recognition. (Methods) 521 subjects yield 1164 slice images via the slice level selection method. All the 1164 slice images comprise four categories: COVID-19 positive; community-acquired pneumonia; second pulmonary tuberculosis; and healthy control. Our method firstly introduced an improved multiple-way data augmentation. Secondly, an n-conv rank-based average pooling module (NRAPM) was proposed in which rank-based pooling—particularly, rank-based average pooling (RAP)—was employed to avoid overfitting. Third, a novel DRAPNet was proposed based on NRAPM and inspired by the VGG network. Grad-CAM was used to generate heatmaps and gave our AI model an explainable analysis. (Results) Our DRAPNet achieved a micro-averaged F1 score of 95.49% by 10 runs over the test set. The sensitivities of the four classes were 95.44%, 96.07%, 94.41%, and 96.07%, respectively. The precisions of four classes were 96.45%, 95.22%, 95.05%, and 95.28%, respectively. The F1 scores of the four classes were 95.94%, 95.64%, 94.73%, and 95.67%, respectively. Besides, the confusion matrix was given. (Conclusions) The DRAPNet is effective in diagnosing COVID-19 and other chest infectious diseases. The RAP gives better results than four other methods: strided convolution, l2-norm pooling, average pooling, and max pooling.Keywords
Cite This Article
Citations
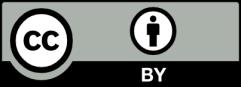
This work is licensed under a Creative Commons Attribution 4.0 International License , which permits unrestricted use, distribution, and reproduction in any medium, provided the original work is properly cited.