Open Access
ARTICLE
Handling Class Imbalance in Online Transaction Fraud Detection
1 School of Computer Science and Engineering, Lovely Professional University, Punjab, India
2 Department of Computing and Mathematics, Manchester Metropolitan University, UK & School of Electrical Engineering and Computer Science, National University of Science and Technology, Islamabad, Pakistan
3 Department of Computer Science and Engineering, Soonchunhyang University, Asan, 31538, Korea
4 Department of Electrical and Computer Engineering, Dhofar University, Salalah, Oman
5 College of Computer Engineering and Sciences, Prince Sattam bin Abdulaziz University, Al-Kharj, 11942, Saudi Arabia
* Corresponding Author: Yunyoung Nam. Email:
(This article belongs to the Special Issue: Emerging Trends in Artificial Intelligence and Machine Learning)
Computers, Materials & Continua 2022, 70(2), 2861-2877. https://doi.org/10.32604/cmc.2022.019990
Received 03 May 2021; Accepted 04 June 2021; Issue published 27 September 2021
Abstract
With the rise of internet facilities, a greater number of people have started doing online transactions at an exponential rate in recent years as the online transaction system has eliminated the need of going to the bank physically for every transaction. However, the fraud cases have also increased causing the loss of money to the consumers. Hence, an effective fraud detection system is the need of the hour which can detect fraudulent transactions automatically in real-time. Generally, the genuine transactions are large in number than the fraudulent transactions which leads to the class imbalance problem. In this research work, an online transaction fraud detection system using deep learning has been proposed which can handle class imbalance problem by applying algorithm-level methods which modify the learning of the model to focus more on the minority class i.e., fraud transactions. A novel loss function named Weighted Hard- Reduced Focal Loss (WH-RFL) has been proposed which has achieved maximum fraud detection rate i.e., True Positive Rate (TPR) at the cost of misclassification of few genuine transactions as high TPR is preferred over a high True Negative Rate (TNR) in fraud detection system and same has been demonstrated using three publicly available imbalanced transactional datasets. Also, Thresholding has been applied to optimize the decision threshold using cross-validation to detect maximum number of frauds and it has been demonstrated by the experimental results that the selection of the right thresholding method with deep learning yields better results.Keywords
Cite This Article
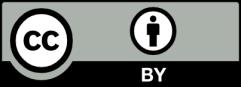
This work is licensed under a Creative Commons Attribution 4.0 International License , which permits unrestricted use, distribution, and reproduction in any medium, provided the original work is properly cited.