Open Access
ARTICLE
User Behavior Traffic Analysis Using a Simplified Memory-Prediction Framework
1 College of Computer Science & IT, Albaha University, Alaqiq, 65779-7738, Saudi Arabia
2 Malaysia School of Information Technology, Monash University, Bandar Sunway, 47500, Malaysia
3 Department of Information Technology, University of Moratuwa, Moratuwa, 10400, Sri Lanka
4 Faculty of Computer Science, Universitas Sriwijaya, Indralaya, 30662, Indonesia
* Corresponding Author: Rahmat Budiarto. Email:
(This article belongs to the Special Issue: Machine Learning Applications in Medical, Finance, Education and Cyber Security)
Computers, Materials & Continua 2022, 70(2), 2679-2698. https://doi.org/10.32604/cmc.2022.019847
Received 28 April 2021; Accepted 15 June 2021; Issue published 27 September 2021
Abstract
As nearly half of the incidents in enterprise security have been triggered by insiders, it is important to deploy a more intelligent defense system to assist enterprises in pinpointing and resolving the incidents caused by insiders or malicious software (malware) in real-time. Failing to do so may cause a serious loss of reputation as well as business. At the same time, modern network traffic has dynamic patterns, high complexity, and large volumes that make it more difficult to detect malware early. The ability to learn tasks sequentially is crucial to the development of artificial intelligence. Existing neurogenetic computation models with deep-learning techniques are able to detect complex patterns; however, the models have limitations, including catastrophic forgetfulness, and require intensive computational resources. As defense systems using deep-learning models require more time to learn new traffic patterns, they cannot perform fully online (on-the-fly) learning. Hence, an intelligent attack/malware detection system with on-the-fly learning capability is required. For this paper, a memory-prediction framework was adopted, and a simplified single cell assembled sequential hierarchical memory (s.SCASHM) model instead of the hierarchical temporal memory (HTM) model is proposed to speed up learning convergence to achieve on-the-fly learning. The s.SCASHM consists of a Single Neuronal Cell (SNC) model and a simplified Sequential Hierarchical Superset (SHS) platform. The s.SCASHM is implemented as the prediction engine of a user behavior analysis tool to detect insider attacks/anomalies. The experimental results show that the proposed memory model can predict users’ traffic behavior with accuracy level ranging from 72% to 83% while performing on-the-fly learning.Keywords
Cite This Article
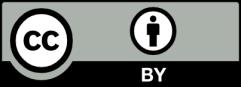
This work is licensed under a Creative Commons Attribution 4.0 International License , which permits unrestricted use, distribution, and reproduction in any medium, provided the original work is properly cited.