Open Access
ARTICLE
Effect of Direct Statistical Contrast Enhancement Technique on Document Image Binarization
1 Advanced Computing (AdvCOMP), Centre of Excellence, Universiti Malaysia Perlis (UniMAP), Pauh Putra Campus, Arau, 02600, Perlis, Malaysia
2 Faculty of Electrical Engineering Technology, Universiti Malaysia Perlis (UniMAP), Pauh Putra Campus, Arau, 02600, Perlis, Malaysia
3 Faculty of Electronic Engineering Technology, Universiti Malaysia Perlis (UniMAP), Pauh Putra Campus, Arau, 02600, Perlis, Malaysia
4 Faculty of Engineering, The Islamic University, Najaf, 54001, Iraq
* Corresponding Author: Wan Azani Mustafa. Email:
Computers, Materials & Continua 2022, 70(2), 3549-3564. https://doi.org/10.32604/cmc.2022.019801
Received 26 April 2021; Accepted 15 June 2021; Issue published 27 September 2021
Abstract
Background: Contrast enhancement plays an important role in the image processing field. Contrast correction has performed an adjustment on the darkness or brightness of the input image and increases the quality of the image. Objective: This paper proposed a novel method based on statistical data from the local mean and local standard deviation. Method: The proposed method modifies the mean and standard deviation of a neighbourhood at each pixel and divides it into three categories: background, foreground, and problematic (contrast & luminosity) region. Experimental results from both visual and objective aspects show that the proposed method can normalize the contrast variation problem effectively compared to Histogram Equalization (HE), Difference of Gaussian (DoG), and Butterworth Homomorphic Filtering (BHF). Seven (7) types of binarization methods were tested on the corrected image and produced a positive and impressive result. Result: Finally, a comparison in terms of Signal Noise Ratio (SNR), Misclassification Error (ME), F-measure, Peak Signal Noise Ratio (PSNR), Misclassification Penalty Metric (MPM), and Accuracy was calculated. Each binarization method shows an incremented result after applying it onto the corrected image compared to the original image. The SNR result of our proposed image is 9.350 higher than the three (3) other methods. The average increment after five (5) types of evaluation are: (Otsu = 41.64%, Local Adaptive = 7.05%, Niblack = 30.28%, Bernsen = 25%, Bradley = 3.54%, Nick = 1.59%, Gradient-Based = 14.6%). Conclusion: The results presented in this paper effectively solve the contrast problem and finally produce better quality images.Keywords
Cite This Article
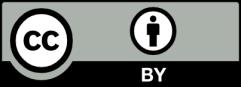