Open Access
ARTICLE
Data Analytics for the Identification of Fake Reviews Using Supervised Learning
1 Department of Computer Science & Information Technology, Dr. Babasaheb Ambedkar Marathwada University, Aurangabad, India
2 Department of Computer Sciences and Information Technology, Albaha University, Saudi Arabia
3 Department of Computer Engineering and Science, Albaha University, Saudi Arabia
4 Community College of Abqaiq, King Faisal University, Al-Ahsa, Saudi Arabia
5 College of Computer Science and Information Technology, King Faisal University, Al-Ahsa, Saudi Arabia
6 Al–Nahrain University, Bagdad, Iraq
* Corresponding Author: Theyazn H. H. Aldhyani. Email:
(This article belongs to the Special Issue: Application of Big Data Analytics in the Management of Business)
Computers, Materials & Continua 2022, 70(2), 3189-3204. https://doi.org/10.32604/cmc.2022.019625
Received 20 April 2021; Accepted 15 June 2021; Issue published 27 September 2021
Abstract
Fake reviews, also known as deceptive opinions, are used to mislead people and have gained more importance recently. This is due to the rapid increase in online marketing transactions, such as selling and purchasing. E-commerce provides a facility for customers to post reviews and comment about the product or service when purchased. New customers usually go through the posted reviews or comments on the website before making a purchase decision. However, the current challenge is how new individuals can distinguish truthful reviews from fake ones, which later deceive customers, inflict losses, and tarnish the reputation of companies. The present paper attempts to develop an intelligent system that can detect fake reviews on ecommerce platforms using n-grams of the review text and sentiment scores given by the reviewer. The proposed methodology adopted in this study used a standard fake hotel review dataset for experimenting and data preprocessing methods and a term frequency-Inverse document frequency (TF-IDF) approach for extracting features and their representation. For detection and classification, n-grams of review texts were inputted into the constructed models to be classified as fake or truthful. However, the experiments were carried out using four different supervised machine-learning techniques and were trained and tested on a dataset collected from the Trip Advisor website. The classification results of these experiments showed that naïve Bayes (NB), support vector machine (SVM), adaptive boosting (AB), and random forest (RF) received 88%, 93%, 94%, and 95%, respectively, based on testing accuracy and the F1-score. The obtained results were compared with existing works that used the same dataset, and the proposed methods outperformed the comparable methods in terms of accuracy.Keywords
Cite This Article
Citations
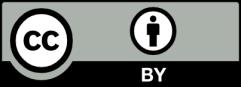