Open Access
ARTICLE
Deep Reinforcement Learning Model for Blood Bank Vehicle Routing Multi-Objective Optimization
1 Director of Advanced Manufacturing and Industry 4.0 Center, King Abdul-Aziz City for Science and Technology Riyadh, Saudi Arabia
2 Data Science and AI Senior Manager Vodafone, Cairo, Egypt
3 Information System Department, Al Imam Mohammad Ibn Saud Islamic University, Riyadh, Saudi Arabia
* Corresponding Author: Meteb M. Altaf. Email:
(This article belongs to the Special Issue: Emerging Trends in Artificial Intelligence and Machine Learning)
Computers, Materials & Continua 2022, 70(2), 3955-3967. https://doi.org/10.32604/cmc.2022.019448
Received 14 April 2021; Accepted 25 June 2021; Issue published 27 September 2021
Abstract
The overall healthcare system has been prioritized within development top lists worldwide. Since many national populations are aging, combined with the availability of sophisticated medical treatments, healthcare expenditures are rapidly growing. Blood banks are a major component of any healthcare system, which store and provide the blood products needed for organ transplants, emergency medical treatments, and routine surgeries. Timely delivery of blood products is vital, especially in emergency settings. Hence, blood delivery process parameters such as safety and speed have received attention in the literature, as well as other parameters such as delivery cost. In this paper, delivery time and cost are modeled mathematically and marked as objective functions requiring simultaneous optimization. A solution is proposed based on Deep Reinforcement Learning (DRL) to address the formulated delivery functions as Multi-objective Optimization Problems (MOPs). The basic concept of the solution is to decompose the MOP into a scalar optimization sub-problems set, where each one of these sub-problems is modeled as a separate Neural Network (NN). The overall model parameters for each sub-problem are optimized based on a neighborhood parameter transfer and DRL training algorithm. The optimization step for the sub-problems is undertaken collaboratively to optimize the overall model. Pareto-optimal solutions can be directly obtained using the trained NN. Specifically, the multi-objective blood bank delivery problem is addressed in this research. One major technical advantage of this approach is that once the trained model is available, it can be scaled without the need for model retraining. The scoring can be obtained directly using a straightforward computation of the NN layers in a limited time. The proposed technique provides a set of technical strength points such as the ability to generalize and solve rapidly compared to other multi-objective optimization methods. The model was trained and tested on 5 major hospitals in Saudi Arabia’s Riyadh region, and the simulation results indicated that time and cost decreased by 35% and 30%, respectively. In particular, the proposed model outperformed other state-of-the-art MOP solutions such as Genetic Algorithms and Simulated Annealing.Keywords
Cite This Article
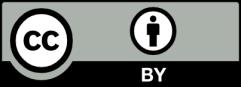
This work is licensed under a Creative Commons Attribution 4.0 International License , which permits unrestricted use, distribution, and reproduction in any medium, provided the original work is properly cited.