Open Access
ARTICLE
A Saliency Based Image Fusion Framework for Skin Lesion Segmentation and Classification
1 Department of Computer Science, COMSATS University Islamabad, Islamabad Campus, Pakistan
2 Department of Electrical and Computer Engineering, COMSATS University Islamabad, Wah Campus, Pakistan
3 Department of Computer Engineering, College of Computer and Information Sciences, King Saud University, Riyadh, 11543, Saudi Arabia
* Corresponding Author: Syed Rameez Naqvi. Email:
(This article belongs to the Special Issue: Recent Advances in Deep Learning for Medical Image Analysis)
Computers, Materials & Continua 2022, 70(2), 3235-3250. https://doi.org/10.32604/cmc.2022.018949
Received 27 March 2021; Accepted 29 May 2021; Issue published 27 September 2021
Abstract
Melanoma, due to its higher mortality rate, is considered as one of the most pernicious types of skin cancers, mostly affecting the white populations. It has been reported a number of times and is now widely accepted, that early detection of melanoma increases the chances of the subject’s survival. Computer-aided diagnostic systems help the experts in diagnosing the skin lesion at earlier stages using machine learning techniques. In this work, we propose a framework that accurately segments, and later classifies, the lesion using improved image segmentation and fusion methods. The proposed technique takes an image and passes it through two methods simultaneously; one is the weighted visual saliency-based method, and the second is improved HDCT based saliency estimation. The resultant image maps are later fused using the proposed image fusion technique to generate a localized lesion region. The resultant binary image is later mapped back to the RGB image and fed into the Inception-ResNet-V2 pre-trained model–trained by applying transfer learning. The simulation results show improved performance compared to several existing methods.Keywords
Cite This Article
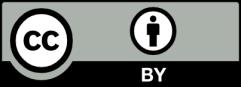
This work is licensed under a Creative Commons Attribution 4.0 International License , which permits unrestricted use, distribution, and reproduction in any medium, provided the original work is properly cited.