Open Access
ARTICLE
Weapons Detection for Security and Video Surveillance Using CNN and YOLO-V5s
1 Department of Computer Science, Shaheed Zulfikar Ali Bhutto Institute of Science and Technology, Islamabad, 44000, Pakistan
2 Department of Computer Science, College of Computers and Information Technology, Taif University, Taif, 21944, Saudi Arabia
3 College of Applied Computer Science, King Saud University (Almuzahmiyah Campus), Riyadh, 11543, Saudi Arabia
4 Department of Computer Science, HITEC University, Taxila, 47080, Pakistan
5 College of Computing and Informatics, Saudi Electronic University, Riyadh, Saudi Arabia
6 Faculty of Engineering, Port Said University, Port Fuad City, Egypt
* Corresponding Author: Abdulrahman M. Qahtani. Email:
Computers, Materials & Continua 2022, 70(2), 2761-2775. https://doi.org/10.32604/cmc.2022.018785
Received 21 March 2021; Accepted 10 May 2021; Issue published 27 September 2021
Abstract
In recent years, the number of Gun-related incidents has crossed over 250,000 per year and over 85% of the existing 1 billion firearms are in civilian hands, manual monitoring has not proven effective in detecting firearms. which is why an automated weapon detection system is needed. Various automated convolutional neural networks (CNN) weapon detection systems have been proposed in the past to generate good results. However, These techniques have high computation overhead and are slow to provide real-time detection which is essential for the weapon detection system. These models have a high rate of false negatives because they often fail to detect the guns due to the low quality and visibility issues of surveillance videos. This research work aims to minimize the rate of false negatives and false positives in weapon detection while keeping the speed of detection as a key parameter. The proposed framework is based on You Only Look Once (YOLO) and Area of Interest (AOI). Initially, the models take pre-processed frames where the background is removed by the use of the Gaussian blur algorithm. The proposed architecture will be assessed through various performance parameters such as False Negative, False Positive, precision, recall rate, and F1 score. The results of this research work make it clear that due to YOLO-v5s high recall rate and speed of detection are achieved. Speed reached 0.010 s per frame compared to the 0.17 s of the Faster R-CNN. It is promising to be used in the field of security and weapon detection.Keywords
Cite This Article
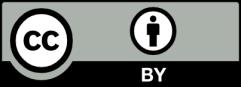
This work is licensed under a Creative Commons Attribution 4.0 International License , which permits unrestricted use, distribution, and reproduction in any medium, provided the original work is properly cited.