Open Access
ARTICLE
Epilepsy Radiology Reports Classification Using Deep Learning Networks
1 Department of Computer Engineering, Halic University, Istanbul, 34445, Turkey
2 Department of Computer Engineering, Istanbul University – Cerrahpasa, Istanbul, 34320, Turkey
3 Department of Computer Engineering, Sivas Cumhuriyet University, Sivas, 58140, Turkey
* Corresponding Author: Eylem Yucel. Email:
(This article belongs to the Special Issue: Machine Learning Applications in Medical, Finance, Education and Cyber Security)
Computers, Materials & Continua 2022, 70(2), 3589-3607. https://doi.org/10.32604/cmc.2022.018742
Received 19 March 2021; Accepted 14 June 2021; Issue published 27 September 2021
Abstract
The automatic and accurate classification of Magnetic Resonance Imaging (MRI) radiology report is essential for the analysis and interpretation epilepsy and non-epilepsy. Since the majority of MRI radiology reports are unstructured, the manual information extraction is time-consuming and requires specific expertise. In this paper, a comprehensive method is proposed to classify epilepsy and non-epilepsy real brain MRI radiology text reports automatically. This method combines the Natural Language Processing technique and statistical Machine Learning methods. 122 real MRI radiology text reports (97 epilepsy, 25 non-epilepsy) are studied by our proposed method which consists of the following steps: (i) for a given text report our systems first cleans HTML/XML tags, tokenize, erase punctuation, normalize text, (ii) then it converts into MRI text reports numeric sequences by using index-based word encoding, (iii) then we applied the deep learning models that are uni-directional long short-term memory (LSTM) network, bidirectional long short-term memory (BiLSTM) network and convolutional neural network (CNN) for the classifying comparison of the data, (iv) finally, we used 70% of used for training, 15% for validation, and 15% for test observations. Unlike previous methods, this study encompasses the following objectives: (a) to extract significant text features from radiologic reports of epilepsy disease; (b) to ensure successful classifying accuracy performance to enhance epilepsy data attributes. Therefore, our study is a comprehensive comparative study with the epilepsy dataset obtained from numeric sequences by using index-based word encoding method applied for the deep learning models. The traditional method is numeric sequences by using index-based word encoding which has been made for the first time in the literature, is successful feature descriptor in the epilepsy data set. The BiLSTM network has shown a promising performance regarding the accuracy rates. We show that the larger sized medical text reports can be analyzed by our proposed method.Keywords
Cite This Article
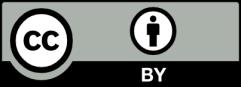
This work is licensed under a Creative Commons Attribution 4.0 International License , which permits unrestricted use, distribution, and reproduction in any medium, provided the original work is properly cited.