Open Access
ARTICLE
Image Segmentation Based on Block Level and Hybrid Directional Local Extrema
1 Manipal University Jaipur, Jaipur, 302026, India
2 BML Munjal University, Gurugram, India
3 Bennett University, Greater Noida, India
4 College of Computer Engineering and Sciences, Prince Sattam Bin Abdulaziz University, Al-Khraj, Saudi Arabia
5 Department of Computer Science, HITEC University Taxila, Taxila, Pakistan
6 Department of ICT Convergence, Soonchunhyang University, Asan 31538, Korea
7 Department of Computer Science and Engineering, Soonchunhyang University, Asan, Korea
* Corresponding Author: Yunyoung Nam. Email:
(This article belongs to the Special Issue: Recent Advances in Deep Learning and Saliency Methods for Agriculture)
Computers, Materials & Continua 2022, 70(2), 3939-3954. https://doi.org/10.32604/cmc.2022.018423
Received 08 March 2021; Accepted 05 May 2021; Issue published 27 September 2021
Abstract
In the recent decade, the digitalization of various tasks has added great flexibility to human lifestyle and has changed daily routine activities of communities. Image segmentation is a key step in digitalization. Segmentation plays a key role in almost all areas of image processing, and various approaches have been proposed for image segmentation. In this paper, a novel approach is proposed for image segmentation using a nonuniform adaptive strategy. Region-based image segmentation along with a directional binary pattern generated a better segmented image. An adaptive mask of 8 × 8 was circulated over the pixels whose bit value was 1 in the generated directional binary pattern. Segmentation was performed in three phases: first, an image was divided into sub-images or image chunks; next, the image patches were taken as input, and an adaptive threshold was generated; and finally the image chunks were processed separately by convolving the adaptive mask on the image chunks. Gradient and Laplacian of Gaussian algorithms along with directional extrema patterns provided a double check for boundary pixels. The proposed approach was tested on chunks of varying sizes, and after multiple iterations, it was found that a block size of 8 × 8 performs better than other chunks or block sizes. The accuracy of the segmentation technique was measured in terms of the count of ill regions, which were extracted after the segmentation process.Keywords
Cite This Article
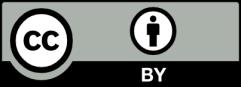
This work is licensed under a Creative Commons Attribution 4.0 International License , which permits unrestricted use, distribution, and reproduction in any medium, provided the original work is properly cited.