Open Access
ARTICLE
Anomaly Based Camera Prioritization in Large Scale Surveillance Networks
1 Department of Software, Sejong University, 143-747, Seoul, Korea
2 Digital Image Processing Lab, Islamia College Peshawar, 25000, Pakistan
3 Color Lab, Department of Computer Science, Norwegian University of Science and Technology (NTNU), 2815, Gjøvik, Norway
4 CORIS Institute, Oregon State University, 97331, Oregon, USA
* Corresponding Author: Muhammad Sajjad. Email:
(This article belongs to the Special Issue: Recent Advances in Deep Learning, Information Fusion, and Features Selection for Video Surveillance Application)
Computers, Materials & Continua 2022, 70(2), 2171-2190. https://doi.org/10.32604/cmc.2022.018181
Received 28 February 2021; Accepted 06 May 2021; Issue published 27 September 2021
Abstract
Digital surveillance systems are ubiquitous and continuously generate massive amounts of data, and manual monitoring is required in order to recognise human activities in public areas. Intelligent surveillance systems that can automatically ide.pngy normal and abnormal activities are highly desirable, as these would allow for efficient monitoring by selecting only those camera feeds in which abnormal activities are occurring. This paper proposes an energy-efficient camera prioritisation framework that intelligently adjusts the priority of cameras in a vast surveillance network using feedback from the activity recognition system. The proposed system addresses the limitations of existing manual monitoring surveillance systems using a three-step framework. In the first step, the salient frames are selected from the online video stream using a frame differencing method. A lightweight 3D convolutional neural network (3DCNN) architecture is applied to extract spatio-temporal features from the salient frames in the second step. Finally, the probabilities predicted by the 3DCNN network and the metadata of the cameras are processed using a linear threshold gate sigmoid mechanism to control the priority of the camera. The proposed system performs well compared to state-of-the-art violent activity recognition methods in terms of efficient camera prioritisation in large-scale surveillance networks. Comprehensive experiments and an evaluation of activity recognition and camera prioritisation showed that our approach achieved an accuracy of 98% with an F1-score of 0.97 on the Hockey Fight dataset, and an accuracy of 99% with an F1-score of 0.98 on the Violent Crowd dataset.Keywords
Cite This Article
Citations
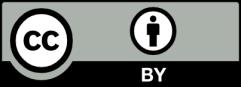