Open Access
ARTICLE
Data Traffic Reduction with Compressed Sensing in an AIoT System
1 Hanshin University, Osan-si, 18101, Korea
2 Hancom with Inc., Seongnam-si, 13493, Korea
* Corresponding Author: Jeongwook Seo. Email:
Computers, Materials & Continua 2022, 70(1), 1769-1780. https://doi.org/10.32604/cmc.2022.020027
Received 06 May 2021; Accepted 07 June 2021; Issue published 07 September 2021
Abstract
To provide Artificial Intelligence (AI) services such as object detection, Internet of Things (IoT) sensor devices should be able to send a large amount of data such as images and videos. However, this inevitably causes IoT networks to be severely overloaded. In this paper, therefore, we propose a novel oneM2M-compliant Artificial Intelligence of Things (AIoT) system for reducing overall data traffic and offering object detection. It consists of some IoT sensor devices with random sampling functions controlled by a compressed sensing (CS) rate, an IoT edge gateway with CS recovery and domain transform functions related to compressed sensing, and a YOLOv5 deep learning function for object detection, and an IoT server. By analyzing the effects of compressed sensing on data traffic reduction in terms of data rate per IoT sensor device, we showed that the proposed AIoT system can reduce the overall data traffic by changing compressed sensing rates of random sampling functions in IoT sensor devices. In addition, we analyzed the effects of the compressed sensing on YOLOv5 object detection in terms of performance metrics such as recall, precision, mAP50, and mAP, and found that recall slightly decreases but precision remains almost constant even though the compressed sensing rate decreases and that mAP50 and mAP are gradually degraded according to the decreased compressed sensing rate. Consequently, if proper compressed sensing rates are chosen, the proposed AIoT system will reduce the overall data traffic without significant performance degradation of YOLOv5.Keywords
Cite This Article
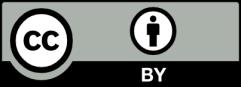