Open Access
ARTICLE
Covid-19 Detection from Chest X-Ray Images Using Advanced Deep Learning Techniques
1 School of Electronics & Communication Engineering, Shri Mata Vaishno Devi University, Katra, 182320, India
2 School of Electrical Engineering, Shri Mata Vaishno Devi University, Katra, 182320, India
3 Department of Mathematics, Faculty of Science, Mansoura University, Mansoura, 35516, Egypt
4 Department of Computational Mathematics, Science, and Engineering (CMSE), Michigan State University, East Lansing, 48824, MI, USA
5 School of Computing, University of Eastern Finland, Kuopio, 70210, Finland
* Corresponding Author: Shubham Mahajan. Email:
Computers, Materials & Continua 2022, 70(1), 1541-1556. https://doi.org/10.32604/cmc.2022.019496
Received 15 April 2021; Accepted 18 May 2021; Issue published 07 September 2021
Abstract
Like the Covid-19 pandemic, smallpox virus infection broke out in the last century, wherein 500 million deaths were reported along with enormous economic loss. But unlike smallpox, the Covid-19 recorded a low exponential infection rate and mortality rate due to advancement in medical aid and diagnostics. Data analytics, machine learning, and automation techniques can help in early diagnostics and supporting treatments of many reported patients. This paper proposes a robust and efficient methodology for the early detection of COVID-19 from Chest X-Ray scans utilizing enhanced deep learning techniques. Our study suggests that using the Prediction and Deconvolutional Modules in combination with the SSD architecture can improve the performance of the model trained at this task. We used a publicly open CXR image dataset and implemented the detection model with task-specific pre-processing and near 80:20 split. This achieved a competitive specificity of 0.9474 and a sensibility/accuracy of 0.9597, which shall help better decision-making for various aspects of identification and treat the infection.Keywords
Cite This Article
Citations
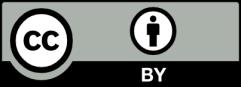