Open Access
ARTICLE
A Position-Aware Transformer for Image Captioning
1 School of Computer and Communication Engineering, Changsha University of Science and Technology, Changsha, 410114, China
2 School of Computer Science and Cyber Engineering, Guangzhou University, Guangzhou, 510006, China
3 Advanced Forming Research Centre, University of Strathclyde, Renfrewshire, PA4 9LJ, Glasgow, United Kingdom
4 Department of Computer Science, Community College, King Saud University, Riyadh, 11437, Saudi Arabia
5 Department of Mathematics and Computer Science, Faculty of Science, Menoufia University, Egypt
* Corresponding Author: Zelin Deng. Email:
Computers, Materials & Continua 2022, 70(1), 2065-2081. https://doi.org/10.32604/cmc.2022.019328
Received 10 April 2021; Accepted 16 June 2021; Issue published 07 September 2021
Abstract
Image captioning aims to generate a corresponding description of an image. In recent years, neural encoder-decoder models have been the dominant approaches, in which the Convolutional Neural Network (CNN) and Long Short Term Memory (LSTM) are used to translate an image into a natural language description. Among these approaches, the visual attention mechanisms are widely used to enable deeper image understanding through fine-grained analysis and even multiple steps of reasoning. However, most conventional visual attention mechanisms are based on high-level image features, ignoring the effects of other image features, and giving insufficient consideration to the relative positions between image features. In this work, we propose a Position-Aware Transformer model with image-feature attention and position-aware attention mechanisms for the above problems. The image-feature attention firstly extracts multi-level features by using Feature Pyramid Network (FPN), then utilizes the scaled-dot-product to fuse these features, which enables our model to detect objects of different scales in the image more effectively without increasing parameters. In the position-aware attention mechanism, the relative positions between image features are obtained at first, afterwards the relative positions are incorporated into the original image features to generate captions more accurately. Experiments are carried out on the MSCOCO dataset and our approach achieves competitive BLEU-4, METEOR, ROUGE-L, CIDEr scores compared with some state-of-the-art approaches, demonstrating the effectiveness of our approach.Keywords
Cite This Article
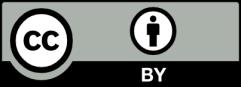
This work is licensed under a Creative Commons Attribution 4.0 International License , which permits unrestricted use, distribution, and reproduction in any medium, provided the original work is properly cited.