Open Access
ARTICLE
Joint Channel and Multi-User Detection Empowered with Machine Learning
1 College of Engineering, Al Ain University, Abu Dhabi, 112612, UAE
2 Department of Computer Science, Lahore Garrison University, Lahore, 54792, Pakistan
3 School of Computer Science, National College of Business Administration and Economics, Lahore, 54000, Pakistan
4 Riphah School of Computing and Innovation, Faculty of Computing, Riphah International University, Lahore, 54000, Pakistan
5 Pattern Recognition and Machine Learning Lab, Department of Software Engineering, Gachon University, Seongnam, 13557, South Korea
6 Department of Computer Science, College of Science and Technology, Wenzhou Kean University, 325060, USA
7 Department of Computer Science, Hameeda Rasheed Institute of Science and Technology, Multan, 66000, Pakistan
* Corresponding Author: Muhammad Adnan Khan. Email:
Computers, Materials & Continua 2022, 70(1), 109-121. https://doi.org/10.32604/cmc.2022.019295
Received 09 April 2021; Accepted 10 May 2021; Issue published 07 September 2021
Abstract
The numbers of multimedia applications and their users increase with each passing day. Different multi-carrier systems have been developed along with varying techniques of space-time coding to address the demand of the future generation of network systems. In this article, a fuzzy logic empowered adaptive backpropagation neural network (FLeABPNN) algorithm is proposed for joint channel and multi-user detection (CMD). FLeABPNN has two stages. The first stage estimates the channel parameters, and the second performs multi-user detection. The proposed approach capitalizes on a neuro-fuzzy hybrid system that combines the competencies of both fuzzy logic and neural networks. This study analyzes the results of using FLeABPNN based on a multiple-input and multiple-output (MIMO) receiver with conventional partial opposite mutant particle swarm optimization (POMPSO), total-OMPSO (TOMPSO), fuzzy logic empowered POMPSO (FL-POMPSO), and FL-TOMPSO-based MIMO receivers. The FLeABPNN-based receiver renders better results than other techniques in terms of minimum mean square error, minimum mean channel error, and bit error rate.Keywords
Cite This Article
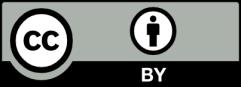
This work is licensed under a Creative Commons Attribution 4.0 International License , which permits unrestricted use, distribution, and reproduction in any medium, provided the original work is properly cited.